Combining Sequential Feature Selection with Grid Search
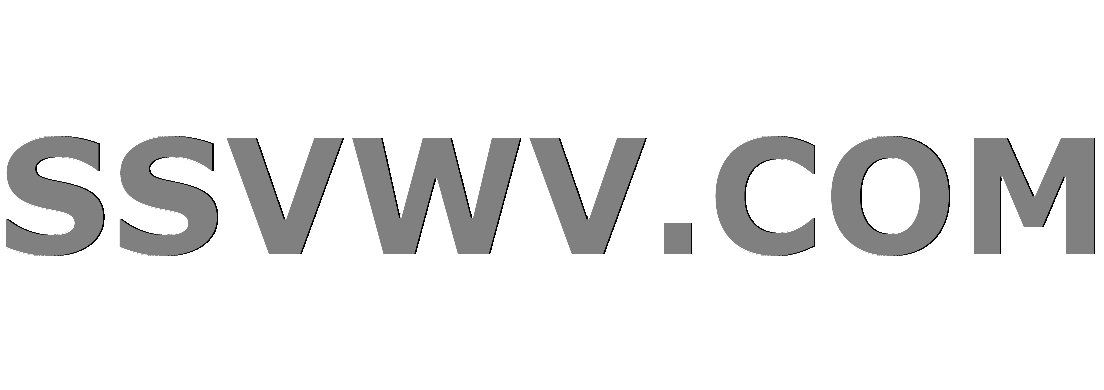
Multi tool use
up vote
0
down vote
favorite
I'm struggling to combine Sequential Feature Selector (from mlxtend) with a GridSearchCV (from sklearn).
My objective is to perform a forward feature selection for each set of parameters, to find which combination of parameters and features produces the best score.
The following code is based on example 8 of the user guide from mlxtend (see http://rasbt.github.io/mlxtend/user_guide/feature_selection/SequentialFeatureSelector/#example-8-sequential-feature-selection-and-gridsearch)
X = data.values #dataframe with 48 features and 200 rows
y = diags #binary classification for each row
X_train, X_test, y_train, y_test = train_test_split(
X, y, test_size=0.3, random_state=0)
svm = svm.SVC()
sfs = SFS(estimator = svm,
k_features = (1,len(data.columns)),
forward = True,
floating=False,
scoring = 'f1',
cv = 5)
pipe = Pipeline([
('sfs', sfs),
('svm', svm)
])
param_grid = [
{
'sfs__estimator__C': [0.001, 0.01, 0.1, 1, 10, 100, 1000],
'sfs__estimator__gamma': ['auto', 'scale', 0.001, 0.0001],
'sfs__estimator__kernel': ['linear', 'rbf'],
}
]
gs = GridSearchCV(estimator = pipe,
param_grid = param_grid,
scoring = 'f1',
n_jobs=1,
cv=5,
refit = True)
gs.fit(X_train, y_train)
print("Best parameters via GridSearch", gs.best_params_)
print("nBest features:n", gs.best_estimator_.steps[0][1].k_feature_idx_)
print("nBest score:n", gs.best_estimator_.steps[0][1].k_score_)
When executing this code I get the following:
Best parameters via GridSearch {'sfs__estimator__gamma': 'auto', 'sfs__estimator__kernel': 'linear', 'sfs__estimator__C': 0.001}
Best features:
(16, 39)
Best score:
0.31333333333333335
I doubted these were the best results as I did actually get better results when setting a higher minimum number of features.
I noticed that changing the order of the grid of parameters modified the results. When using:
param_grid = [
{
# Notice the change of order for C, 0.01 is now first
'sfs__estimator__C': [0.01, 0.001, 0.1, 1, 10, 100, 1000],
'sfs__estimator__gamma': ['auto', 'scale', 0.001, 0.0001],
'sfs__estimator__kernel': ['linear', 'rbf'],
}
]
I got the following results:
Best parameters via GridSearch {'sfs__estimator__gamma': 'auto', 'sfs__estimator__kernel': 'linear', 'sfs__estimator__C': 0.01}
Best features:
(16, 39)
Best score:
0.4428571428571429
Best parameters seem to always return the first value of each parameter. I tried other combinations and the parameters where always the first ones, and the best features did't change.
Am I using GridSearchCV wrong? Or am I printing the wrong attributes?
python scikit-learn svm grid-search mlxtend
add a comment |
up vote
0
down vote
favorite
I'm struggling to combine Sequential Feature Selector (from mlxtend) with a GridSearchCV (from sklearn).
My objective is to perform a forward feature selection for each set of parameters, to find which combination of parameters and features produces the best score.
The following code is based on example 8 of the user guide from mlxtend (see http://rasbt.github.io/mlxtend/user_guide/feature_selection/SequentialFeatureSelector/#example-8-sequential-feature-selection-and-gridsearch)
X = data.values #dataframe with 48 features and 200 rows
y = diags #binary classification for each row
X_train, X_test, y_train, y_test = train_test_split(
X, y, test_size=0.3, random_state=0)
svm = svm.SVC()
sfs = SFS(estimator = svm,
k_features = (1,len(data.columns)),
forward = True,
floating=False,
scoring = 'f1',
cv = 5)
pipe = Pipeline([
('sfs', sfs),
('svm', svm)
])
param_grid = [
{
'sfs__estimator__C': [0.001, 0.01, 0.1, 1, 10, 100, 1000],
'sfs__estimator__gamma': ['auto', 'scale', 0.001, 0.0001],
'sfs__estimator__kernel': ['linear', 'rbf'],
}
]
gs = GridSearchCV(estimator = pipe,
param_grid = param_grid,
scoring = 'f1',
n_jobs=1,
cv=5,
refit = True)
gs.fit(X_train, y_train)
print("Best parameters via GridSearch", gs.best_params_)
print("nBest features:n", gs.best_estimator_.steps[0][1].k_feature_idx_)
print("nBest score:n", gs.best_estimator_.steps[0][1].k_score_)
When executing this code I get the following:
Best parameters via GridSearch {'sfs__estimator__gamma': 'auto', 'sfs__estimator__kernel': 'linear', 'sfs__estimator__C': 0.001}
Best features:
(16, 39)
Best score:
0.31333333333333335
I doubted these were the best results as I did actually get better results when setting a higher minimum number of features.
I noticed that changing the order of the grid of parameters modified the results. When using:
param_grid = [
{
# Notice the change of order for C, 0.01 is now first
'sfs__estimator__C': [0.01, 0.001, 0.1, 1, 10, 100, 1000],
'sfs__estimator__gamma': ['auto', 'scale', 0.001, 0.0001],
'sfs__estimator__kernel': ['linear', 'rbf'],
}
]
I got the following results:
Best parameters via GridSearch {'sfs__estimator__gamma': 'auto', 'sfs__estimator__kernel': 'linear', 'sfs__estimator__C': 0.01}
Best features:
(16, 39)
Best score:
0.4428571428571429
Best parameters seem to always return the first value of each parameter. I tried other combinations and the parameters where always the first ones, and the best features did't change.
Am I using GridSearchCV wrong? Or am I printing the wrong attributes?
python scikit-learn svm grid-search mlxtend
add a comment |
up vote
0
down vote
favorite
up vote
0
down vote
favorite
I'm struggling to combine Sequential Feature Selector (from mlxtend) with a GridSearchCV (from sklearn).
My objective is to perform a forward feature selection for each set of parameters, to find which combination of parameters and features produces the best score.
The following code is based on example 8 of the user guide from mlxtend (see http://rasbt.github.io/mlxtend/user_guide/feature_selection/SequentialFeatureSelector/#example-8-sequential-feature-selection-and-gridsearch)
X = data.values #dataframe with 48 features and 200 rows
y = diags #binary classification for each row
X_train, X_test, y_train, y_test = train_test_split(
X, y, test_size=0.3, random_state=0)
svm = svm.SVC()
sfs = SFS(estimator = svm,
k_features = (1,len(data.columns)),
forward = True,
floating=False,
scoring = 'f1',
cv = 5)
pipe = Pipeline([
('sfs', sfs),
('svm', svm)
])
param_grid = [
{
'sfs__estimator__C': [0.001, 0.01, 0.1, 1, 10, 100, 1000],
'sfs__estimator__gamma': ['auto', 'scale', 0.001, 0.0001],
'sfs__estimator__kernel': ['linear', 'rbf'],
}
]
gs = GridSearchCV(estimator = pipe,
param_grid = param_grid,
scoring = 'f1',
n_jobs=1,
cv=5,
refit = True)
gs.fit(X_train, y_train)
print("Best parameters via GridSearch", gs.best_params_)
print("nBest features:n", gs.best_estimator_.steps[0][1].k_feature_idx_)
print("nBest score:n", gs.best_estimator_.steps[0][1].k_score_)
When executing this code I get the following:
Best parameters via GridSearch {'sfs__estimator__gamma': 'auto', 'sfs__estimator__kernel': 'linear', 'sfs__estimator__C': 0.001}
Best features:
(16, 39)
Best score:
0.31333333333333335
I doubted these were the best results as I did actually get better results when setting a higher minimum number of features.
I noticed that changing the order of the grid of parameters modified the results. When using:
param_grid = [
{
# Notice the change of order for C, 0.01 is now first
'sfs__estimator__C': [0.01, 0.001, 0.1, 1, 10, 100, 1000],
'sfs__estimator__gamma': ['auto', 'scale', 0.001, 0.0001],
'sfs__estimator__kernel': ['linear', 'rbf'],
}
]
I got the following results:
Best parameters via GridSearch {'sfs__estimator__gamma': 'auto', 'sfs__estimator__kernel': 'linear', 'sfs__estimator__C': 0.01}
Best features:
(16, 39)
Best score:
0.4428571428571429
Best parameters seem to always return the first value of each parameter. I tried other combinations and the parameters where always the first ones, and the best features did't change.
Am I using GridSearchCV wrong? Or am I printing the wrong attributes?
python scikit-learn svm grid-search mlxtend
I'm struggling to combine Sequential Feature Selector (from mlxtend) with a GridSearchCV (from sklearn).
My objective is to perform a forward feature selection for each set of parameters, to find which combination of parameters and features produces the best score.
The following code is based on example 8 of the user guide from mlxtend (see http://rasbt.github.io/mlxtend/user_guide/feature_selection/SequentialFeatureSelector/#example-8-sequential-feature-selection-and-gridsearch)
X = data.values #dataframe with 48 features and 200 rows
y = diags #binary classification for each row
X_train, X_test, y_train, y_test = train_test_split(
X, y, test_size=0.3, random_state=0)
svm = svm.SVC()
sfs = SFS(estimator = svm,
k_features = (1,len(data.columns)),
forward = True,
floating=False,
scoring = 'f1',
cv = 5)
pipe = Pipeline([
('sfs', sfs),
('svm', svm)
])
param_grid = [
{
'sfs__estimator__C': [0.001, 0.01, 0.1, 1, 10, 100, 1000],
'sfs__estimator__gamma': ['auto', 'scale', 0.001, 0.0001],
'sfs__estimator__kernel': ['linear', 'rbf'],
}
]
gs = GridSearchCV(estimator = pipe,
param_grid = param_grid,
scoring = 'f1',
n_jobs=1,
cv=5,
refit = True)
gs.fit(X_train, y_train)
print("Best parameters via GridSearch", gs.best_params_)
print("nBest features:n", gs.best_estimator_.steps[0][1].k_feature_idx_)
print("nBest score:n", gs.best_estimator_.steps[0][1].k_score_)
When executing this code I get the following:
Best parameters via GridSearch {'sfs__estimator__gamma': 'auto', 'sfs__estimator__kernel': 'linear', 'sfs__estimator__C': 0.001}
Best features:
(16, 39)
Best score:
0.31333333333333335
I doubted these were the best results as I did actually get better results when setting a higher minimum number of features.
I noticed that changing the order of the grid of parameters modified the results. When using:
param_grid = [
{
# Notice the change of order for C, 0.01 is now first
'sfs__estimator__C': [0.01, 0.001, 0.1, 1, 10, 100, 1000],
'sfs__estimator__gamma': ['auto', 'scale', 0.001, 0.0001],
'sfs__estimator__kernel': ['linear', 'rbf'],
}
]
I got the following results:
Best parameters via GridSearch {'sfs__estimator__gamma': 'auto', 'sfs__estimator__kernel': 'linear', 'sfs__estimator__C': 0.01}
Best features:
(16, 39)
Best score:
0.4428571428571429
Best parameters seem to always return the first value of each parameter. I tried other combinations and the parameters where always the first ones, and the best features did't change.
Am I using GridSearchCV wrong? Or am I printing the wrong attributes?
python scikit-learn svm grid-search mlxtend
python scikit-learn svm grid-search mlxtend
asked Nov 22 at 11:35


Eric R
12
12
add a comment |
add a comment |
active
oldest
votes
Your Answer
StackExchange.ifUsing("editor", function () {
StackExchange.using("externalEditor", function () {
StackExchange.using("snippets", function () {
StackExchange.snippets.init();
});
});
}, "code-snippets");
StackExchange.ready(function() {
var channelOptions = {
tags: "".split(" "),
id: "1"
};
initTagRenderer("".split(" "), "".split(" "), channelOptions);
StackExchange.using("externalEditor", function() {
// Have to fire editor after snippets, if snippets enabled
if (StackExchange.settings.snippets.snippetsEnabled) {
StackExchange.using("snippets", function() {
createEditor();
});
}
else {
createEditor();
}
});
function createEditor() {
StackExchange.prepareEditor({
heartbeatType: 'answer',
convertImagesToLinks: true,
noModals: true,
showLowRepImageUploadWarning: true,
reputationToPostImages: 10,
bindNavPrevention: true,
postfix: "",
imageUploader: {
brandingHtml: "Powered by u003ca class="icon-imgur-white" href="https://imgur.com/"u003eu003c/au003e",
contentPolicyHtml: "User contributions licensed under u003ca href="https://creativecommons.org/licenses/by-sa/3.0/"u003ecc by-sa 3.0 with attribution requiredu003c/au003e u003ca href="https://stackoverflow.com/legal/content-policy"u003e(content policy)u003c/au003e",
allowUrls: true
},
onDemand: true,
discardSelector: ".discard-answer"
,immediatelyShowMarkdownHelp:true
});
}
});
Sign up or log in
StackExchange.ready(function () {
StackExchange.helpers.onClickDraftSave('#login-link');
});
Sign up using Google
Sign up using Facebook
Sign up using Email and Password
Post as a guest
Required, but never shown
StackExchange.ready(
function () {
StackExchange.openid.initPostLogin('.new-post-login', 'https%3a%2f%2fstackoverflow.com%2fquestions%2f53430101%2fcombining-sequential-feature-selection-with-grid-search%23new-answer', 'question_page');
}
);
Post as a guest
Required, but never shown
active
oldest
votes
active
oldest
votes
active
oldest
votes
active
oldest
votes
Thanks for contributing an answer to Stack Overflow!
- Please be sure to answer the question. Provide details and share your research!
But avoid …
- Asking for help, clarification, or responding to other answers.
- Making statements based on opinion; back them up with references or personal experience.
To learn more, see our tips on writing great answers.
Some of your past answers have not been well-received, and you're in danger of being blocked from answering.
Please pay close attention to the following guidance:
- Please be sure to answer the question. Provide details and share your research!
But avoid …
- Asking for help, clarification, or responding to other answers.
- Making statements based on opinion; back them up with references or personal experience.
To learn more, see our tips on writing great answers.
Sign up or log in
StackExchange.ready(function () {
StackExchange.helpers.onClickDraftSave('#login-link');
});
Sign up using Google
Sign up using Facebook
Sign up using Email and Password
Post as a guest
Required, but never shown
StackExchange.ready(
function () {
StackExchange.openid.initPostLogin('.new-post-login', 'https%3a%2f%2fstackoverflow.com%2fquestions%2f53430101%2fcombining-sequential-feature-selection-with-grid-search%23new-answer', 'question_page');
}
);
Post as a guest
Required, but never shown
Sign up or log in
StackExchange.ready(function () {
StackExchange.helpers.onClickDraftSave('#login-link');
});
Sign up using Google
Sign up using Facebook
Sign up using Email and Password
Post as a guest
Required, but never shown
Sign up or log in
StackExchange.ready(function () {
StackExchange.helpers.onClickDraftSave('#login-link');
});
Sign up using Google
Sign up using Facebook
Sign up using Email and Password
Post as a guest
Required, but never shown
Sign up or log in
StackExchange.ready(function () {
StackExchange.helpers.onClickDraftSave('#login-link');
});
Sign up using Google
Sign up using Facebook
Sign up using Email and Password
Sign up using Google
Sign up using Facebook
Sign up using Email and Password
Post as a guest
Required, but never shown
Required, but never shown
Required, but never shown
Required, but never shown
Required, but never shown
Required, but never shown
Required, but never shown
Required, but never shown
Required, but never shown
nLCNAXgm2LXMiXUhWVG6,RPKeYrvD632V7kNGK C 468c9a