Using sklearn precision_recall_curve function with different classifiers
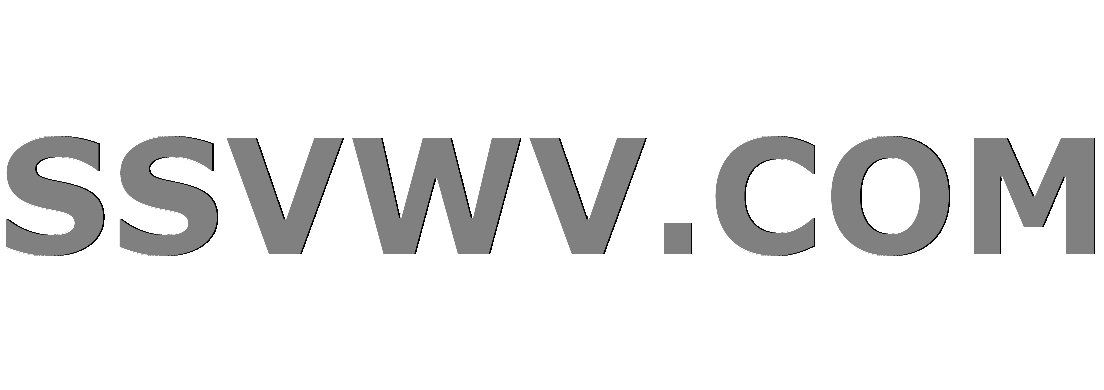
Multi tool use
up vote
0
down vote
favorite
This may be an easy question, but I need help understanding how to use the precision_recall_curve function in sklearn.
I have a binary dataset, and am using three classifiers (SVM, RF, LR) to classify it.
The example in sklearn's documentation shows to use the function like this:
y_score = classifier.decision_function(X_test)
precision_recall_curve(y_test, y_score)
In the example, "decision_function" is a built in function for SVM classifiers. However, I don't see a function like that for Random Forest classifiers or Linear Regression.
Can someone help me understand what the y_score and decision function really is, and how I can calculate it for any classifier?
Thanks!
python scikit-learn precision precision-recall
add a comment |
up vote
0
down vote
favorite
This may be an easy question, but I need help understanding how to use the precision_recall_curve function in sklearn.
I have a binary dataset, and am using three classifiers (SVM, RF, LR) to classify it.
The example in sklearn's documentation shows to use the function like this:
y_score = classifier.decision_function(X_test)
precision_recall_curve(y_test, y_score)
In the example, "decision_function" is a built in function for SVM classifiers. However, I don't see a function like that for Random Forest classifiers or Linear Regression.
Can someone help me understand what the y_score and decision function really is, and how I can calculate it for any classifier?
Thanks!
python scikit-learn precision precision-recall
add a comment |
up vote
0
down vote
favorite
up vote
0
down vote
favorite
This may be an easy question, but I need help understanding how to use the precision_recall_curve function in sklearn.
I have a binary dataset, and am using three classifiers (SVM, RF, LR) to classify it.
The example in sklearn's documentation shows to use the function like this:
y_score = classifier.decision_function(X_test)
precision_recall_curve(y_test, y_score)
In the example, "decision_function" is a built in function for SVM classifiers. However, I don't see a function like that for Random Forest classifiers or Linear Regression.
Can someone help me understand what the y_score and decision function really is, and how I can calculate it for any classifier?
Thanks!
python scikit-learn precision precision-recall
This may be an easy question, but I need help understanding how to use the precision_recall_curve function in sklearn.
I have a binary dataset, and am using three classifiers (SVM, RF, LR) to classify it.
The example in sklearn's documentation shows to use the function like this:
y_score = classifier.decision_function(X_test)
precision_recall_curve(y_test, y_score)
In the example, "decision_function" is a built in function for SVM classifiers. However, I don't see a function like that for Random Forest classifiers or Linear Regression.
Can someone help me understand what the y_score and decision function really is, and how I can calculate it for any classifier?
Thanks!
python scikit-learn precision precision-recall
python scikit-learn precision precision-recall
edited Nov 22 at 13:45


Gabriel M
1,09841223
1,09841223
asked Nov 22 at 12:55
Zofia
1
1
add a comment |
add a comment |
2 Answers
2
active
oldest
votes
up vote
0
down vote
Look at the second param description in documentation of precision_recall_curve
:
probas_pred : array, shape = [n_samples]
Estimated probabilities or decision function.
When decision_function()
is not present, you may use predict_proba()
in its place.
add a comment |
up vote
0
down vote
For all the other classifiers that do not have a built in decision_function
,
you shall use the predict_proba
function, that does esentially the same thing.
y_score = random_forest.predict_proba()
add a comment |
Your Answer
StackExchange.ifUsing("editor", function () {
StackExchange.using("externalEditor", function () {
StackExchange.using("snippets", function () {
StackExchange.snippets.init();
});
});
}, "code-snippets");
StackExchange.ready(function() {
var channelOptions = {
tags: "".split(" "),
id: "1"
};
initTagRenderer("".split(" "), "".split(" "), channelOptions);
StackExchange.using("externalEditor", function() {
// Have to fire editor after snippets, if snippets enabled
if (StackExchange.settings.snippets.snippetsEnabled) {
StackExchange.using("snippets", function() {
createEditor();
});
}
else {
createEditor();
}
});
function createEditor() {
StackExchange.prepareEditor({
heartbeatType: 'answer',
convertImagesToLinks: true,
noModals: true,
showLowRepImageUploadWarning: true,
reputationToPostImages: 10,
bindNavPrevention: true,
postfix: "",
imageUploader: {
brandingHtml: "Powered by u003ca class="icon-imgur-white" href="https://imgur.com/"u003eu003c/au003e",
contentPolicyHtml: "User contributions licensed under u003ca href="https://creativecommons.org/licenses/by-sa/3.0/"u003ecc by-sa 3.0 with attribution requiredu003c/au003e u003ca href="https://stackoverflow.com/legal/content-policy"u003e(content policy)u003c/au003e",
allowUrls: true
},
onDemand: true,
discardSelector: ".discard-answer"
,immediatelyShowMarkdownHelp:true
});
}
});
Sign up or log in
StackExchange.ready(function () {
StackExchange.helpers.onClickDraftSave('#login-link');
});
Sign up using Google
Sign up using Facebook
Sign up using Email and Password
Post as a guest
Required, but never shown
StackExchange.ready(
function () {
StackExchange.openid.initPostLogin('.new-post-login', 'https%3a%2f%2fstackoverflow.com%2fquestions%2f53431525%2fusing-sklearn-precision-recall-curve-function-with-different-classifiers%23new-answer', 'question_page');
}
);
Post as a guest
Required, but never shown
2 Answers
2
active
oldest
votes
2 Answers
2
active
oldest
votes
active
oldest
votes
active
oldest
votes
up vote
0
down vote
Look at the second param description in documentation of precision_recall_curve
:
probas_pred : array, shape = [n_samples]
Estimated probabilities or decision function.
When decision_function()
is not present, you may use predict_proba()
in its place.
add a comment |
up vote
0
down vote
Look at the second param description in documentation of precision_recall_curve
:
probas_pred : array, shape = [n_samples]
Estimated probabilities or decision function.
When decision_function()
is not present, you may use predict_proba()
in its place.
add a comment |
up vote
0
down vote
up vote
0
down vote
Look at the second param description in documentation of precision_recall_curve
:
probas_pred : array, shape = [n_samples]
Estimated probabilities or decision function.
When decision_function()
is not present, you may use predict_proba()
in its place.
Look at the second param description in documentation of precision_recall_curve
:
probas_pred : array, shape = [n_samples]
Estimated probabilities or decision function.
When decision_function()
is not present, you may use predict_proba()
in its place.
answered Nov 22 at 13:00
Vivek Kumar
14.7k41850
14.7k41850
add a comment |
add a comment |
up vote
0
down vote
For all the other classifiers that do not have a built in decision_function
,
you shall use the predict_proba
function, that does esentially the same thing.
y_score = random_forest.predict_proba()
add a comment |
up vote
0
down vote
For all the other classifiers that do not have a built in decision_function
,
you shall use the predict_proba
function, that does esentially the same thing.
y_score = random_forest.predict_proba()
add a comment |
up vote
0
down vote
up vote
0
down vote
For all the other classifiers that do not have a built in decision_function
,
you shall use the predict_proba
function, that does esentially the same thing.
y_score = random_forest.predict_proba()
For all the other classifiers that do not have a built in decision_function
,
you shall use the predict_proba
function, that does esentially the same thing.
y_score = random_forest.predict_proba()
answered Nov 22 at 13:00


Gabriel M
1,09841223
1,09841223
add a comment |
add a comment |
Thanks for contributing an answer to Stack Overflow!
- Please be sure to answer the question. Provide details and share your research!
But avoid …
- Asking for help, clarification, or responding to other answers.
- Making statements based on opinion; back them up with references or personal experience.
To learn more, see our tips on writing great answers.
Some of your past answers have not been well-received, and you're in danger of being blocked from answering.
Please pay close attention to the following guidance:
- Please be sure to answer the question. Provide details and share your research!
But avoid …
- Asking for help, clarification, or responding to other answers.
- Making statements based on opinion; back them up with references or personal experience.
To learn more, see our tips on writing great answers.
Sign up or log in
StackExchange.ready(function () {
StackExchange.helpers.onClickDraftSave('#login-link');
});
Sign up using Google
Sign up using Facebook
Sign up using Email and Password
Post as a guest
Required, but never shown
StackExchange.ready(
function () {
StackExchange.openid.initPostLogin('.new-post-login', 'https%3a%2f%2fstackoverflow.com%2fquestions%2f53431525%2fusing-sklearn-precision-recall-curve-function-with-different-classifiers%23new-answer', 'question_page');
}
);
Post as a guest
Required, but never shown
Sign up or log in
StackExchange.ready(function () {
StackExchange.helpers.onClickDraftSave('#login-link');
});
Sign up using Google
Sign up using Facebook
Sign up using Email and Password
Post as a guest
Required, but never shown
Sign up or log in
StackExchange.ready(function () {
StackExchange.helpers.onClickDraftSave('#login-link');
});
Sign up using Google
Sign up using Facebook
Sign up using Email and Password
Post as a guest
Required, but never shown
Sign up or log in
StackExchange.ready(function () {
StackExchange.helpers.onClickDraftSave('#login-link');
});
Sign up using Google
Sign up using Facebook
Sign up using Email and Password
Sign up using Google
Sign up using Facebook
Sign up using Email and Password
Post as a guest
Required, but never shown
Required, but never shown
Required, but never shown
Required, but never shown
Required, but never shown
Required, but never shown
Required, but never shown
Required, but never shown
Required, but never shown
MAz17mga