How to transform structured streams with PySpark?
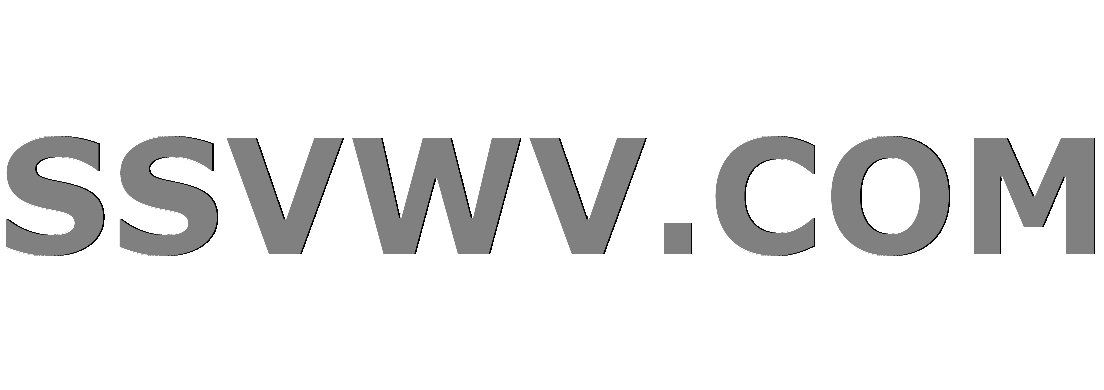
Multi tool use
This seems like it should be obvious, but in reviewing the docs and examples, I'm not sure I can find a way to take a structured stream and transform using PySpark.
For example:
from pyspark.sql import SparkSession
spark = (
SparkSession
.builder
.appName('StreamingWordCount')
.getOrCreate()
)
raw_records = (
spark
.readStream
.format('socket')
.option('host', 'localhost')
.option('port', 9999)
.load()
)
# I realize there's a SQL function for upper-case, just illustrating a sample
# use of an arbitrary map function
records = raw_records.rdd.map(lambda w: w.upper()).toDF()
counts = (
records
.groupBy(records.value)
.count()
)
query = (
counts
.writeStream
.outputMode('complete')
.format('console')
.start()
)
query.awaitTermination()
This will throw the following exception:
Queries with streaming sources must be executed with writeStream.start
However, if I remove the call to rdd.map(...).toDF()
things seem to work fine.
Seems as though the call to rdd.map
branched execution from the streaming context and causes Spark to warn that it was never started?
Is there a "right" way to apply map
or mapPartition
style transformations using Structured Streaming and PySpark?
apache-spark pyspark spark-structured-streaming
add a comment |
This seems like it should be obvious, but in reviewing the docs and examples, I'm not sure I can find a way to take a structured stream and transform using PySpark.
For example:
from pyspark.sql import SparkSession
spark = (
SparkSession
.builder
.appName('StreamingWordCount')
.getOrCreate()
)
raw_records = (
spark
.readStream
.format('socket')
.option('host', 'localhost')
.option('port', 9999)
.load()
)
# I realize there's a SQL function for upper-case, just illustrating a sample
# use of an arbitrary map function
records = raw_records.rdd.map(lambda w: w.upper()).toDF()
counts = (
records
.groupBy(records.value)
.count()
)
query = (
counts
.writeStream
.outputMode('complete')
.format('console')
.start()
)
query.awaitTermination()
This will throw the following exception:
Queries with streaming sources must be executed with writeStream.start
However, if I remove the call to rdd.map(...).toDF()
things seem to work fine.
Seems as though the call to rdd.map
branched execution from the streaming context and causes Spark to warn that it was never started?
Is there a "right" way to apply map
or mapPartition
style transformations using Structured Streaming and PySpark?
apache-spark pyspark spark-structured-streaming
add a comment |
This seems like it should be obvious, but in reviewing the docs and examples, I'm not sure I can find a way to take a structured stream and transform using PySpark.
For example:
from pyspark.sql import SparkSession
spark = (
SparkSession
.builder
.appName('StreamingWordCount')
.getOrCreate()
)
raw_records = (
spark
.readStream
.format('socket')
.option('host', 'localhost')
.option('port', 9999)
.load()
)
# I realize there's a SQL function for upper-case, just illustrating a sample
# use of an arbitrary map function
records = raw_records.rdd.map(lambda w: w.upper()).toDF()
counts = (
records
.groupBy(records.value)
.count()
)
query = (
counts
.writeStream
.outputMode('complete')
.format('console')
.start()
)
query.awaitTermination()
This will throw the following exception:
Queries with streaming sources must be executed with writeStream.start
However, if I remove the call to rdd.map(...).toDF()
things seem to work fine.
Seems as though the call to rdd.map
branched execution from the streaming context and causes Spark to warn that it was never started?
Is there a "right" way to apply map
or mapPartition
style transformations using Structured Streaming and PySpark?
apache-spark pyspark spark-structured-streaming
This seems like it should be obvious, but in reviewing the docs and examples, I'm not sure I can find a way to take a structured stream and transform using PySpark.
For example:
from pyspark.sql import SparkSession
spark = (
SparkSession
.builder
.appName('StreamingWordCount')
.getOrCreate()
)
raw_records = (
spark
.readStream
.format('socket')
.option('host', 'localhost')
.option('port', 9999)
.load()
)
# I realize there's a SQL function for upper-case, just illustrating a sample
# use of an arbitrary map function
records = raw_records.rdd.map(lambda w: w.upper()).toDF()
counts = (
records
.groupBy(records.value)
.count()
)
query = (
counts
.writeStream
.outputMode('complete')
.format('console')
.start()
)
query.awaitTermination()
This will throw the following exception:
Queries with streaming sources must be executed with writeStream.start
However, if I remove the call to rdd.map(...).toDF()
things seem to work fine.
Seems as though the call to rdd.map
branched execution from the streaming context and causes Spark to warn that it was never started?
Is there a "right" way to apply map
or mapPartition
style transformations using Structured Streaming and PySpark?
apache-spark pyspark spark-structured-streaming
apache-spark pyspark spark-structured-streaming
edited Oct 28 '18 at 11:33
user6910411
32.8k86995
32.8k86995
asked Jul 25 '18 at 17:56
Mike Sukmanowsky
5532820
5532820
add a comment |
add a comment |
1 Answer
1
active
oldest
votes
Every transformation that is applied in Structured Streaming has to be fully contained in Dataset
world - in case of PySpark it means you can use only DataFrame
or SQL and conversion to RDD
(or DStream
or local collections) are not supported.
If you want to use plain Python code you have to use UserDefinedFunction
.
from pyspark.sql.functions import udf
@udf
def to_upper(s)
return s.upper()
raw_records.select(to_upper("value"))
See also Spark Structured Streaming and Spark-Ml Regression
add a comment |
Your Answer
StackExchange.ifUsing("editor", function () {
StackExchange.using("externalEditor", function () {
StackExchange.using("snippets", function () {
StackExchange.snippets.init();
});
});
}, "code-snippets");
StackExchange.ready(function() {
var channelOptions = {
tags: "".split(" "),
id: "1"
};
initTagRenderer("".split(" "), "".split(" "), channelOptions);
StackExchange.using("externalEditor", function() {
// Have to fire editor after snippets, if snippets enabled
if (StackExchange.settings.snippets.snippetsEnabled) {
StackExchange.using("snippets", function() {
createEditor();
});
}
else {
createEditor();
}
});
function createEditor() {
StackExchange.prepareEditor({
heartbeatType: 'answer',
autoActivateHeartbeat: false,
convertImagesToLinks: true,
noModals: true,
showLowRepImageUploadWarning: true,
reputationToPostImages: 10,
bindNavPrevention: true,
postfix: "",
imageUploader: {
brandingHtml: "Powered by u003ca class="icon-imgur-white" href="https://imgur.com/"u003eu003c/au003e",
contentPolicyHtml: "User contributions licensed under u003ca href="https://creativecommons.org/licenses/by-sa/3.0/"u003ecc by-sa 3.0 with attribution requiredu003c/au003e u003ca href="https://stackoverflow.com/legal/content-policy"u003e(content policy)u003c/au003e",
allowUrls: true
},
onDemand: true,
discardSelector: ".discard-answer"
,immediatelyShowMarkdownHelp:true
});
}
});
Sign up or log in
StackExchange.ready(function () {
StackExchange.helpers.onClickDraftSave('#login-link');
});
Sign up using Google
Sign up using Facebook
Sign up using Email and Password
Post as a guest
Required, but never shown
StackExchange.ready(
function () {
StackExchange.openid.initPostLogin('.new-post-login', 'https%3a%2f%2fstackoverflow.com%2fquestions%2f51525042%2fhow-to-transform-structured-streams-with-pyspark%23new-answer', 'question_page');
}
);
Post as a guest
Required, but never shown
1 Answer
1
active
oldest
votes
1 Answer
1
active
oldest
votes
active
oldest
votes
active
oldest
votes
Every transformation that is applied in Structured Streaming has to be fully contained in Dataset
world - in case of PySpark it means you can use only DataFrame
or SQL and conversion to RDD
(or DStream
or local collections) are not supported.
If you want to use plain Python code you have to use UserDefinedFunction
.
from pyspark.sql.functions import udf
@udf
def to_upper(s)
return s.upper()
raw_records.select(to_upper("value"))
See also Spark Structured Streaming and Spark-Ml Regression
add a comment |
Every transformation that is applied in Structured Streaming has to be fully contained in Dataset
world - in case of PySpark it means you can use only DataFrame
or SQL and conversion to RDD
(or DStream
or local collections) are not supported.
If you want to use plain Python code you have to use UserDefinedFunction
.
from pyspark.sql.functions import udf
@udf
def to_upper(s)
return s.upper()
raw_records.select(to_upper("value"))
See also Spark Structured Streaming and Spark-Ml Regression
add a comment |
Every transformation that is applied in Structured Streaming has to be fully contained in Dataset
world - in case of PySpark it means you can use only DataFrame
or SQL and conversion to RDD
(or DStream
or local collections) are not supported.
If you want to use plain Python code you have to use UserDefinedFunction
.
from pyspark.sql.functions import udf
@udf
def to_upper(s)
return s.upper()
raw_records.select(to_upper("value"))
See also Spark Structured Streaming and Spark-Ml Regression
Every transformation that is applied in Structured Streaming has to be fully contained in Dataset
world - in case of PySpark it means you can use only DataFrame
or SQL and conversion to RDD
(or DStream
or local collections) are not supported.
If you want to use plain Python code you have to use UserDefinedFunction
.
from pyspark.sql.functions import udf
@udf
def to_upper(s)
return s.upper()
raw_records.select(to_upper("value"))
See also Spark Structured Streaming and Spark-Ml Regression
edited Nov 23 '18 at 17:22
user6910411
32.8k86995
32.8k86995
answered Jul 25 '18 at 21:36
user10135885
561
561
add a comment |
add a comment |
Thanks for contributing an answer to Stack Overflow!
- Please be sure to answer the question. Provide details and share your research!
But avoid …
- Asking for help, clarification, or responding to other answers.
- Making statements based on opinion; back them up with references or personal experience.
To learn more, see our tips on writing great answers.
Some of your past answers have not been well-received, and you're in danger of being blocked from answering.
Please pay close attention to the following guidance:
- Please be sure to answer the question. Provide details and share your research!
But avoid …
- Asking for help, clarification, or responding to other answers.
- Making statements based on opinion; back them up with references or personal experience.
To learn more, see our tips on writing great answers.
Sign up or log in
StackExchange.ready(function () {
StackExchange.helpers.onClickDraftSave('#login-link');
});
Sign up using Google
Sign up using Facebook
Sign up using Email and Password
Post as a guest
Required, but never shown
StackExchange.ready(
function () {
StackExchange.openid.initPostLogin('.new-post-login', 'https%3a%2f%2fstackoverflow.com%2fquestions%2f51525042%2fhow-to-transform-structured-streams-with-pyspark%23new-answer', 'question_page');
}
);
Post as a guest
Required, but never shown
Sign up or log in
StackExchange.ready(function () {
StackExchange.helpers.onClickDraftSave('#login-link');
});
Sign up using Google
Sign up using Facebook
Sign up using Email and Password
Post as a guest
Required, but never shown
Sign up or log in
StackExchange.ready(function () {
StackExchange.helpers.onClickDraftSave('#login-link');
});
Sign up using Google
Sign up using Facebook
Sign up using Email and Password
Post as a guest
Required, but never shown
Sign up or log in
StackExchange.ready(function () {
StackExchange.helpers.onClickDraftSave('#login-link');
});
Sign up using Google
Sign up using Facebook
Sign up using Email and Password
Sign up using Google
Sign up using Facebook
Sign up using Email and Password
Post as a guest
Required, but never shown
Required, but never shown
Required, but never shown
Required, but never shown
Required, but never shown
Required, but never shown
Required, but never shown
Required, but never shown
Required, but never shown
dQ3IguI3yhKJnSC Oz1It6Lrs dcw