Relationship between a categorical variable and a Bernoulli variable in R
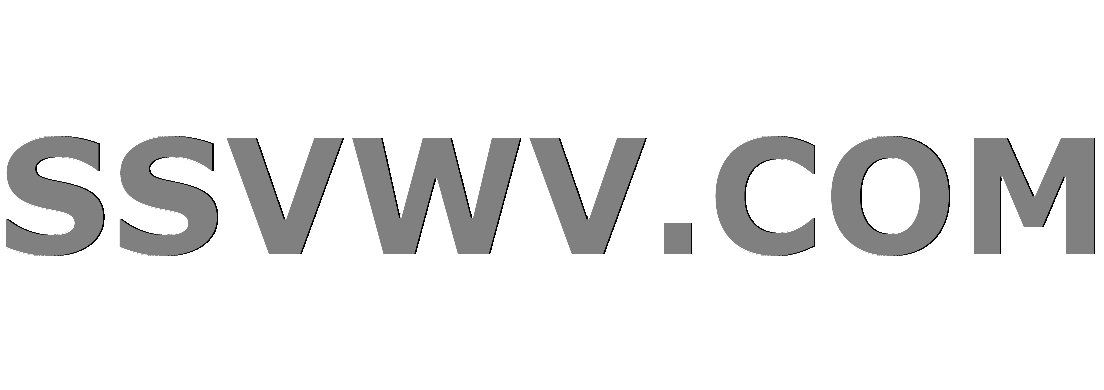
Multi tool use
I would like to answer the following question:
What is the relationship between race and the likelihood of filing a state claim?
Race is a categorical variable but since the response (State_Claim_Made) is 0,1, I'm not exactly sure the best way to go about answering this question without a logistic model.
Below is my code for State_Claim_Made prediction over time (Convicted) which works perfectly.
ggplot(jail, aes(x=Convicted, y=State_Claim_Made)) +
geom_point() +
geom_smooth(method = "glm",
method.args = list(family = "binomial"),
se = TRUE)
I am struggling however to translate this same idea to a categorical variable (Race) and it's relationship to the likelihood of filing a state claim (State_Claim_Made).
DATA SAMPLE:
structure(list(Last_Name = c("Banks", "Beamon", "Dandridge",
"Deakle, Jr.", "Doyle", "Drinkard", "Ellis", "Embry", "Gaines",
"Gurley", "Hinton", "Holemon", "Holsomback", "Hunt", "Jones",
"Mahan", "Mahan", "McMillian", "Moore", "Padgett"), First_Name = c("Medell",
"Melvin Todd", "Beniah Alton", "Evan Lee", "Robert E.", "Gary",
"Andre", "Anthony", "Freddie Lee", "Timothy", "Anthony", "Jeffrey",
"John", "H. Guy", "Lydia Diane", "Dale", "Ronnie", "Walter",
"Daniel Wade", "Larry Randal"), Age = c("27", "24", "29", "59",
"44", "37", "35", "23", "22", "22", "29", "23", "33", "54", "40",
"22", "26", "45", "24", "40"), Race = c("Black", "Black", "Caucasian",
"Caucasian", "Caucasian", "Caucasian", "Black", "Black", "Black",
"Caucasian", "Black", "Caucasian", "Caucasian", "Caucasian",
"Black", "Caucasian", "Caucasian", "Black", "Caucasian", "Caucasian"
), Sex = c("Male", "Male", "Male", "Male", "Male", "Male", "Male",
"Male", "Male", "Male", "Male", "Male", "Male", "Male", "Female",
"Male", "Male", "Male", "Male", "Male"), State = c("Alabama",
"Alabama", "Alabama", "Alabama", "Alabama", "Alabama", "Alabama",
"Alabama", "Alabama", "Alabama", "Alabama", "Alabama", "Alabama",
"Alabama", "Alabama", "Alabama", "Alabama", "Alabama", "Alabama",
"Alabama"), CIU = c(0, 0, 1, 0, 0, 0, 0, 1, 0, 0, 0, 1, 1, 0,
0, 0, 0, 0, 1, 0), Guilty_Plea = c(1, 0, 0, 0, 0, 0, 0, 1, 0,
0, 0, 0, 0, 0, 0, 0, 0, 0, 0, 0), IO = c(0, 0, 0, 0, 0, 0, 0,
0, 0, 0, 0, 0, 0, 0, 0, 0, 0, 0, 0, 0), Worst_Crime = c(6, 1,
1, 4, 4, 1, 2, 1, 1, 6, 1, 2, 4, 6, 3, 2, 2, 1, 1, 1), Occurred = c(1999,
1988, 1994, 2014, 1991, 1993, 2012, 1992, 1972, 1999, 1985, 1987,
1987, 1987, 1997, 1983, 1983, 1986, 1999, 1990), Convicted = c(2001,
1989, 1996, 2015, 1992, 1995, 2013, 1993, 1974, 2000, 1986, 1988,
1988, 1993, 2000, 1986, 1986, 1988, 2002, 1992), Exonerated = c(2003,
1990, 2015, 2015, 2001, 2001, 2014, 1997, 1991, 2002, 2015, 1999,
2000, 1998, 2006, 1998, 1998, 1993, 2009, 1997), Sentence = c("15",
"25", "Life", "Not sentenced", "20", "Death", "85", "20", "30",
"35", "Death", "Life", "25", "Probation", "Life without parole",
"35", "Life without parole", "Death", "Death", "Death"), Death_Penalty = c(0,
0, 0, 0, 0, 1, 0, 0, 0, 0, 1, 0, 0, 0, 0, 0, 0, 1, 1, 1), DNA_Only = c(0,
0, 0, 0, 0, 0, 0, 0, 0, 0, 0, 1, 0, 0, 0, 1, 1, 0, 0, 0), FC = c(1,
0, 0, 0, 0, 0, 0, 0, 0, 0, 0, 0, 0, 0, 0, 0, 0, 0, 0, 0), MWID = c(0,
0, 0, 0, 0, 0, 0, 1, 1, 1, 1, 1, 0, 0, 0, 1, 1, 0, 0, 0), F_MFE = c(0,
0, 1, 0, 0, 0, 0, 0, 0, 0, 1, 0, 0, 0, 0, 0, 0, 0, 0, 1), P_FA = c(1,
1, 1, 1, 1, 0, 1, 0, 0, 0, 0, 0, 1, 0, 0, 0, 0, 1, 0, 0), OM = c(1,
1, 1, 0, 1, 0, 1, 0, 0, 0, 0, 0, 0, 0, 0, 0, 0, 1, 1, 1), ILD = c(0,
0, 1, 0, 0, 1, 0, 0, 0, 0, 1, 0, 1, 0, 1, 0, 0, 0, 0, 0), State_Statute = c("Y",
"Y", "Y", "Y", "Y", "Y", "Y", "Y", "Y", "Y", "Y", "Y", "Y", "Y",
"Y", "Y", "Y", "Y", "Y", "Y"), State_Claim_Made = c(0, 0, 1,
0, 1, 0, 1, 0, 1, 0, 1, 0, 0, 0, 1, 0, 1, 0, 1 0), Zero_time = c(0,
0, 0, 1, 0, 0, 0, 0, 0, 0, 0, 0, 0, 1, 0, 0, 0, 0, 0, 0), Prem = c(0,
0, 0, 0, 0, 0, 0, 0, 0, 0, 0, 0, 0, 0, 0, 0, 0, 0, 0, 0), Pending = c(0,
0, 1, 0, 0, 0, 0, 0, 0, 0, 1, 0, 0, 0, 0, 0, 0, 0, 0, 0), Denied = c(0,
0, 0, 0, 0, 0, 1, 0, 0, 0, 0, 0, 0, 0, 0, 0, 0, 0, 0, 0), State_Award = c("0",
"0", "2", "0", "1", "0", "0", "0", "1", "0", "2", "0", "0", "0",
"0", "0", "0", "0", "0", "0"), Amount = c("0", "0", NA, "0",
"129041.88", "0", "0", "0", "1000000", "0", NA, "0", "0", "0",
"0", "0", "0", "0", "0", "0"), `Non-Statutory_Case_Filed` = c(0,
0, 0, 0, 0, 0, 0, 0, 0, 0, 0, 0, 0, 0, 0, 0, 0, 1, 1, 0), No_Time = c(0,
0, 0, 1, 0, 0, 0, 0, 0, 0, 0, 0, 0, 1, 0, 0, 0, 0, 0, 0), Unfiled = c(1,
1, 1, 1, 1, 1, 1, 1, 1, 1, 1, 1, 1, 1, 1, 1, 1, 0, 0, 1), Dismissed = c(0,
0, 0, 0, 0, 0, 0, 0, 0, 0, 0, 0, 0, 0, 0, 0, 0, 0, 1, 0), Pending__1 = c(0,
0, 0, 0, 0, 0, 0, 0, 0, 0, 0, 0, 0, 0, 0, 0, 0, 0, 0, 0), Award = c(0,
0, 0, 0, 0, 0, 0, 0, 0, 0, 0, 0, 0, 0, 0, 0, 0, 1, 0, 0), Premature = c(0,
0, 0, 0, 0, 0, 0, 0, 0, 0, 0, 0, 0, 0, 0, 0, 0, 0, 0, 0), Amount__1 = c("0",
"0", "0", "0", "0", "0", "0", "0", "0", "0", "0", "0", "0", "0",
"0", "0", "0", "$ undisclosed", "0", "0"), Years_Lost = c(1.7,
0.1, 19.5, 0, 2.6, 5.7, 1.8, 4, 10.7, 1.5, 28.5, 10.6, 10.1,
0, 5.8, 11.4, 11.4, 4.5, 5.4, 5.5), State_Award2 = c("0", "0",
"0", "0", "1", "0", "0", "0", "1", "0", "0", "0", "0", "0", "0",
"0", "0", "0", "0", "0")), row.names = c(NA, -20L), class = c("tbl_df",
"tbl", "data.frame"))
r ggplot2 categorical-data
migrated from stackoverflow.com Nov 24 '18 at 10:20
This question came from our site for professional and enthusiast programmers.
add a comment |
I would like to answer the following question:
What is the relationship between race and the likelihood of filing a state claim?
Race is a categorical variable but since the response (State_Claim_Made) is 0,1, I'm not exactly sure the best way to go about answering this question without a logistic model.
Below is my code for State_Claim_Made prediction over time (Convicted) which works perfectly.
ggplot(jail, aes(x=Convicted, y=State_Claim_Made)) +
geom_point() +
geom_smooth(method = "glm",
method.args = list(family = "binomial"),
se = TRUE)
I am struggling however to translate this same idea to a categorical variable (Race) and it's relationship to the likelihood of filing a state claim (State_Claim_Made).
DATA SAMPLE:
structure(list(Last_Name = c("Banks", "Beamon", "Dandridge",
"Deakle, Jr.", "Doyle", "Drinkard", "Ellis", "Embry", "Gaines",
"Gurley", "Hinton", "Holemon", "Holsomback", "Hunt", "Jones",
"Mahan", "Mahan", "McMillian", "Moore", "Padgett"), First_Name = c("Medell",
"Melvin Todd", "Beniah Alton", "Evan Lee", "Robert E.", "Gary",
"Andre", "Anthony", "Freddie Lee", "Timothy", "Anthony", "Jeffrey",
"John", "H. Guy", "Lydia Diane", "Dale", "Ronnie", "Walter",
"Daniel Wade", "Larry Randal"), Age = c("27", "24", "29", "59",
"44", "37", "35", "23", "22", "22", "29", "23", "33", "54", "40",
"22", "26", "45", "24", "40"), Race = c("Black", "Black", "Caucasian",
"Caucasian", "Caucasian", "Caucasian", "Black", "Black", "Black",
"Caucasian", "Black", "Caucasian", "Caucasian", "Caucasian",
"Black", "Caucasian", "Caucasian", "Black", "Caucasian", "Caucasian"
), Sex = c("Male", "Male", "Male", "Male", "Male", "Male", "Male",
"Male", "Male", "Male", "Male", "Male", "Male", "Male", "Female",
"Male", "Male", "Male", "Male", "Male"), State = c("Alabama",
"Alabama", "Alabama", "Alabama", "Alabama", "Alabama", "Alabama",
"Alabama", "Alabama", "Alabama", "Alabama", "Alabama", "Alabama",
"Alabama", "Alabama", "Alabama", "Alabama", "Alabama", "Alabama",
"Alabama"), CIU = c(0, 0, 1, 0, 0, 0, 0, 1, 0, 0, 0, 1, 1, 0,
0, 0, 0, 0, 1, 0), Guilty_Plea = c(1, 0, 0, 0, 0, 0, 0, 1, 0,
0, 0, 0, 0, 0, 0, 0, 0, 0, 0, 0), IO = c(0, 0, 0, 0, 0, 0, 0,
0, 0, 0, 0, 0, 0, 0, 0, 0, 0, 0, 0, 0), Worst_Crime = c(6, 1,
1, 4, 4, 1, 2, 1, 1, 6, 1, 2, 4, 6, 3, 2, 2, 1, 1, 1), Occurred = c(1999,
1988, 1994, 2014, 1991, 1993, 2012, 1992, 1972, 1999, 1985, 1987,
1987, 1987, 1997, 1983, 1983, 1986, 1999, 1990), Convicted = c(2001,
1989, 1996, 2015, 1992, 1995, 2013, 1993, 1974, 2000, 1986, 1988,
1988, 1993, 2000, 1986, 1986, 1988, 2002, 1992), Exonerated = c(2003,
1990, 2015, 2015, 2001, 2001, 2014, 1997, 1991, 2002, 2015, 1999,
2000, 1998, 2006, 1998, 1998, 1993, 2009, 1997), Sentence = c("15",
"25", "Life", "Not sentenced", "20", "Death", "85", "20", "30",
"35", "Death", "Life", "25", "Probation", "Life without parole",
"35", "Life without parole", "Death", "Death", "Death"), Death_Penalty = c(0,
0, 0, 0, 0, 1, 0, 0, 0, 0, 1, 0, 0, 0, 0, 0, 0, 1, 1, 1), DNA_Only = c(0,
0, 0, 0, 0, 0, 0, 0, 0, 0, 0, 1, 0, 0, 0, 1, 1, 0, 0, 0), FC = c(1,
0, 0, 0, 0, 0, 0, 0, 0, 0, 0, 0, 0, 0, 0, 0, 0, 0, 0, 0), MWID = c(0,
0, 0, 0, 0, 0, 0, 1, 1, 1, 1, 1, 0, 0, 0, 1, 1, 0, 0, 0), F_MFE = c(0,
0, 1, 0, 0, 0, 0, 0, 0, 0, 1, 0, 0, 0, 0, 0, 0, 0, 0, 1), P_FA = c(1,
1, 1, 1, 1, 0, 1, 0, 0, 0, 0, 0, 1, 0, 0, 0, 0, 1, 0, 0), OM = c(1,
1, 1, 0, 1, 0, 1, 0, 0, 0, 0, 0, 0, 0, 0, 0, 0, 1, 1, 1), ILD = c(0,
0, 1, 0, 0, 1, 0, 0, 0, 0, 1, 0, 1, 0, 1, 0, 0, 0, 0, 0), State_Statute = c("Y",
"Y", "Y", "Y", "Y", "Y", "Y", "Y", "Y", "Y", "Y", "Y", "Y", "Y",
"Y", "Y", "Y", "Y", "Y", "Y"), State_Claim_Made = c(0, 0, 1,
0, 1, 0, 1, 0, 1, 0, 1, 0, 0, 0, 1, 0, 1, 0, 1 0), Zero_time = c(0,
0, 0, 1, 0, 0, 0, 0, 0, 0, 0, 0, 0, 1, 0, 0, 0, 0, 0, 0), Prem = c(0,
0, 0, 0, 0, 0, 0, 0, 0, 0, 0, 0, 0, 0, 0, 0, 0, 0, 0, 0), Pending = c(0,
0, 1, 0, 0, 0, 0, 0, 0, 0, 1, 0, 0, 0, 0, 0, 0, 0, 0, 0), Denied = c(0,
0, 0, 0, 0, 0, 1, 0, 0, 0, 0, 0, 0, 0, 0, 0, 0, 0, 0, 0), State_Award = c("0",
"0", "2", "0", "1", "0", "0", "0", "1", "0", "2", "0", "0", "0",
"0", "0", "0", "0", "0", "0"), Amount = c("0", "0", NA, "0",
"129041.88", "0", "0", "0", "1000000", "0", NA, "0", "0", "0",
"0", "0", "0", "0", "0", "0"), `Non-Statutory_Case_Filed` = c(0,
0, 0, 0, 0, 0, 0, 0, 0, 0, 0, 0, 0, 0, 0, 0, 0, 1, 1, 0), No_Time = c(0,
0, 0, 1, 0, 0, 0, 0, 0, 0, 0, 0, 0, 1, 0, 0, 0, 0, 0, 0), Unfiled = c(1,
1, 1, 1, 1, 1, 1, 1, 1, 1, 1, 1, 1, 1, 1, 1, 1, 0, 0, 1), Dismissed = c(0,
0, 0, 0, 0, 0, 0, 0, 0, 0, 0, 0, 0, 0, 0, 0, 0, 0, 1, 0), Pending__1 = c(0,
0, 0, 0, 0, 0, 0, 0, 0, 0, 0, 0, 0, 0, 0, 0, 0, 0, 0, 0), Award = c(0,
0, 0, 0, 0, 0, 0, 0, 0, 0, 0, 0, 0, 0, 0, 0, 0, 1, 0, 0), Premature = c(0,
0, 0, 0, 0, 0, 0, 0, 0, 0, 0, 0, 0, 0, 0, 0, 0, 0, 0, 0), Amount__1 = c("0",
"0", "0", "0", "0", "0", "0", "0", "0", "0", "0", "0", "0", "0",
"0", "0", "0", "$ undisclosed", "0", "0"), Years_Lost = c(1.7,
0.1, 19.5, 0, 2.6, 5.7, 1.8, 4, 10.7, 1.5, 28.5, 10.6, 10.1,
0, 5.8, 11.4, 11.4, 4.5, 5.4, 5.5), State_Award2 = c("0", "0",
"0", "0", "1", "0", "0", "0", "1", "0", "0", "0", "0", "0", "0",
"0", "0", "0", "0", "0")), row.names = c(NA, -20L), class = c("tbl_df",
"tbl", "data.frame"))
r ggplot2 categorical-data
migrated from stackoverflow.com Nov 24 '18 at 10:20
This question came from our site for professional and enthusiast programmers.
add a comment |
I would like to answer the following question:
What is the relationship between race and the likelihood of filing a state claim?
Race is a categorical variable but since the response (State_Claim_Made) is 0,1, I'm not exactly sure the best way to go about answering this question without a logistic model.
Below is my code for State_Claim_Made prediction over time (Convicted) which works perfectly.
ggplot(jail, aes(x=Convicted, y=State_Claim_Made)) +
geom_point() +
geom_smooth(method = "glm",
method.args = list(family = "binomial"),
se = TRUE)
I am struggling however to translate this same idea to a categorical variable (Race) and it's relationship to the likelihood of filing a state claim (State_Claim_Made).
DATA SAMPLE:
structure(list(Last_Name = c("Banks", "Beamon", "Dandridge",
"Deakle, Jr.", "Doyle", "Drinkard", "Ellis", "Embry", "Gaines",
"Gurley", "Hinton", "Holemon", "Holsomback", "Hunt", "Jones",
"Mahan", "Mahan", "McMillian", "Moore", "Padgett"), First_Name = c("Medell",
"Melvin Todd", "Beniah Alton", "Evan Lee", "Robert E.", "Gary",
"Andre", "Anthony", "Freddie Lee", "Timothy", "Anthony", "Jeffrey",
"John", "H. Guy", "Lydia Diane", "Dale", "Ronnie", "Walter",
"Daniel Wade", "Larry Randal"), Age = c("27", "24", "29", "59",
"44", "37", "35", "23", "22", "22", "29", "23", "33", "54", "40",
"22", "26", "45", "24", "40"), Race = c("Black", "Black", "Caucasian",
"Caucasian", "Caucasian", "Caucasian", "Black", "Black", "Black",
"Caucasian", "Black", "Caucasian", "Caucasian", "Caucasian",
"Black", "Caucasian", "Caucasian", "Black", "Caucasian", "Caucasian"
), Sex = c("Male", "Male", "Male", "Male", "Male", "Male", "Male",
"Male", "Male", "Male", "Male", "Male", "Male", "Male", "Female",
"Male", "Male", "Male", "Male", "Male"), State = c("Alabama",
"Alabama", "Alabama", "Alabama", "Alabama", "Alabama", "Alabama",
"Alabama", "Alabama", "Alabama", "Alabama", "Alabama", "Alabama",
"Alabama", "Alabama", "Alabama", "Alabama", "Alabama", "Alabama",
"Alabama"), CIU = c(0, 0, 1, 0, 0, 0, 0, 1, 0, 0, 0, 1, 1, 0,
0, 0, 0, 0, 1, 0), Guilty_Plea = c(1, 0, 0, 0, 0, 0, 0, 1, 0,
0, 0, 0, 0, 0, 0, 0, 0, 0, 0, 0), IO = c(0, 0, 0, 0, 0, 0, 0,
0, 0, 0, 0, 0, 0, 0, 0, 0, 0, 0, 0, 0), Worst_Crime = c(6, 1,
1, 4, 4, 1, 2, 1, 1, 6, 1, 2, 4, 6, 3, 2, 2, 1, 1, 1), Occurred = c(1999,
1988, 1994, 2014, 1991, 1993, 2012, 1992, 1972, 1999, 1985, 1987,
1987, 1987, 1997, 1983, 1983, 1986, 1999, 1990), Convicted = c(2001,
1989, 1996, 2015, 1992, 1995, 2013, 1993, 1974, 2000, 1986, 1988,
1988, 1993, 2000, 1986, 1986, 1988, 2002, 1992), Exonerated = c(2003,
1990, 2015, 2015, 2001, 2001, 2014, 1997, 1991, 2002, 2015, 1999,
2000, 1998, 2006, 1998, 1998, 1993, 2009, 1997), Sentence = c("15",
"25", "Life", "Not sentenced", "20", "Death", "85", "20", "30",
"35", "Death", "Life", "25", "Probation", "Life without parole",
"35", "Life without parole", "Death", "Death", "Death"), Death_Penalty = c(0,
0, 0, 0, 0, 1, 0, 0, 0, 0, 1, 0, 0, 0, 0, 0, 0, 1, 1, 1), DNA_Only = c(0,
0, 0, 0, 0, 0, 0, 0, 0, 0, 0, 1, 0, 0, 0, 1, 1, 0, 0, 0), FC = c(1,
0, 0, 0, 0, 0, 0, 0, 0, 0, 0, 0, 0, 0, 0, 0, 0, 0, 0, 0), MWID = c(0,
0, 0, 0, 0, 0, 0, 1, 1, 1, 1, 1, 0, 0, 0, 1, 1, 0, 0, 0), F_MFE = c(0,
0, 1, 0, 0, 0, 0, 0, 0, 0, 1, 0, 0, 0, 0, 0, 0, 0, 0, 1), P_FA = c(1,
1, 1, 1, 1, 0, 1, 0, 0, 0, 0, 0, 1, 0, 0, 0, 0, 1, 0, 0), OM = c(1,
1, 1, 0, 1, 0, 1, 0, 0, 0, 0, 0, 0, 0, 0, 0, 0, 1, 1, 1), ILD = c(0,
0, 1, 0, 0, 1, 0, 0, 0, 0, 1, 0, 1, 0, 1, 0, 0, 0, 0, 0), State_Statute = c("Y",
"Y", "Y", "Y", "Y", "Y", "Y", "Y", "Y", "Y", "Y", "Y", "Y", "Y",
"Y", "Y", "Y", "Y", "Y", "Y"), State_Claim_Made = c(0, 0, 1,
0, 1, 0, 1, 0, 1, 0, 1, 0, 0, 0, 1, 0, 1, 0, 1 0), Zero_time = c(0,
0, 0, 1, 0, 0, 0, 0, 0, 0, 0, 0, 0, 1, 0, 0, 0, 0, 0, 0), Prem = c(0,
0, 0, 0, 0, 0, 0, 0, 0, 0, 0, 0, 0, 0, 0, 0, 0, 0, 0, 0), Pending = c(0,
0, 1, 0, 0, 0, 0, 0, 0, 0, 1, 0, 0, 0, 0, 0, 0, 0, 0, 0), Denied = c(0,
0, 0, 0, 0, 0, 1, 0, 0, 0, 0, 0, 0, 0, 0, 0, 0, 0, 0, 0), State_Award = c("0",
"0", "2", "0", "1", "0", "0", "0", "1", "0", "2", "0", "0", "0",
"0", "0", "0", "0", "0", "0"), Amount = c("0", "0", NA, "0",
"129041.88", "0", "0", "0", "1000000", "0", NA, "0", "0", "0",
"0", "0", "0", "0", "0", "0"), `Non-Statutory_Case_Filed` = c(0,
0, 0, 0, 0, 0, 0, 0, 0, 0, 0, 0, 0, 0, 0, 0, 0, 1, 1, 0), No_Time = c(0,
0, 0, 1, 0, 0, 0, 0, 0, 0, 0, 0, 0, 1, 0, 0, 0, 0, 0, 0), Unfiled = c(1,
1, 1, 1, 1, 1, 1, 1, 1, 1, 1, 1, 1, 1, 1, 1, 1, 0, 0, 1), Dismissed = c(0,
0, 0, 0, 0, 0, 0, 0, 0, 0, 0, 0, 0, 0, 0, 0, 0, 0, 1, 0), Pending__1 = c(0,
0, 0, 0, 0, 0, 0, 0, 0, 0, 0, 0, 0, 0, 0, 0, 0, 0, 0, 0), Award = c(0,
0, 0, 0, 0, 0, 0, 0, 0, 0, 0, 0, 0, 0, 0, 0, 0, 1, 0, 0), Premature = c(0,
0, 0, 0, 0, 0, 0, 0, 0, 0, 0, 0, 0, 0, 0, 0, 0, 0, 0, 0), Amount__1 = c("0",
"0", "0", "0", "0", "0", "0", "0", "0", "0", "0", "0", "0", "0",
"0", "0", "0", "$ undisclosed", "0", "0"), Years_Lost = c(1.7,
0.1, 19.5, 0, 2.6, 5.7, 1.8, 4, 10.7, 1.5, 28.5, 10.6, 10.1,
0, 5.8, 11.4, 11.4, 4.5, 5.4, 5.5), State_Award2 = c("0", "0",
"0", "0", "1", "0", "0", "0", "1", "0", "0", "0", "0", "0", "0",
"0", "0", "0", "0", "0")), row.names = c(NA, -20L), class = c("tbl_df",
"tbl", "data.frame"))
r ggplot2 categorical-data
I would like to answer the following question:
What is the relationship between race and the likelihood of filing a state claim?
Race is a categorical variable but since the response (State_Claim_Made) is 0,1, I'm not exactly sure the best way to go about answering this question without a logistic model.
Below is my code for State_Claim_Made prediction over time (Convicted) which works perfectly.
ggplot(jail, aes(x=Convicted, y=State_Claim_Made)) +
geom_point() +
geom_smooth(method = "glm",
method.args = list(family = "binomial"),
se = TRUE)
I am struggling however to translate this same idea to a categorical variable (Race) and it's relationship to the likelihood of filing a state claim (State_Claim_Made).
DATA SAMPLE:
structure(list(Last_Name = c("Banks", "Beamon", "Dandridge",
"Deakle, Jr.", "Doyle", "Drinkard", "Ellis", "Embry", "Gaines",
"Gurley", "Hinton", "Holemon", "Holsomback", "Hunt", "Jones",
"Mahan", "Mahan", "McMillian", "Moore", "Padgett"), First_Name = c("Medell",
"Melvin Todd", "Beniah Alton", "Evan Lee", "Robert E.", "Gary",
"Andre", "Anthony", "Freddie Lee", "Timothy", "Anthony", "Jeffrey",
"John", "H. Guy", "Lydia Diane", "Dale", "Ronnie", "Walter",
"Daniel Wade", "Larry Randal"), Age = c("27", "24", "29", "59",
"44", "37", "35", "23", "22", "22", "29", "23", "33", "54", "40",
"22", "26", "45", "24", "40"), Race = c("Black", "Black", "Caucasian",
"Caucasian", "Caucasian", "Caucasian", "Black", "Black", "Black",
"Caucasian", "Black", "Caucasian", "Caucasian", "Caucasian",
"Black", "Caucasian", "Caucasian", "Black", "Caucasian", "Caucasian"
), Sex = c("Male", "Male", "Male", "Male", "Male", "Male", "Male",
"Male", "Male", "Male", "Male", "Male", "Male", "Male", "Female",
"Male", "Male", "Male", "Male", "Male"), State = c("Alabama",
"Alabama", "Alabama", "Alabama", "Alabama", "Alabama", "Alabama",
"Alabama", "Alabama", "Alabama", "Alabama", "Alabama", "Alabama",
"Alabama", "Alabama", "Alabama", "Alabama", "Alabama", "Alabama",
"Alabama"), CIU = c(0, 0, 1, 0, 0, 0, 0, 1, 0, 0, 0, 1, 1, 0,
0, 0, 0, 0, 1, 0), Guilty_Plea = c(1, 0, 0, 0, 0, 0, 0, 1, 0,
0, 0, 0, 0, 0, 0, 0, 0, 0, 0, 0), IO = c(0, 0, 0, 0, 0, 0, 0,
0, 0, 0, 0, 0, 0, 0, 0, 0, 0, 0, 0, 0), Worst_Crime = c(6, 1,
1, 4, 4, 1, 2, 1, 1, 6, 1, 2, 4, 6, 3, 2, 2, 1, 1, 1), Occurred = c(1999,
1988, 1994, 2014, 1991, 1993, 2012, 1992, 1972, 1999, 1985, 1987,
1987, 1987, 1997, 1983, 1983, 1986, 1999, 1990), Convicted = c(2001,
1989, 1996, 2015, 1992, 1995, 2013, 1993, 1974, 2000, 1986, 1988,
1988, 1993, 2000, 1986, 1986, 1988, 2002, 1992), Exonerated = c(2003,
1990, 2015, 2015, 2001, 2001, 2014, 1997, 1991, 2002, 2015, 1999,
2000, 1998, 2006, 1998, 1998, 1993, 2009, 1997), Sentence = c("15",
"25", "Life", "Not sentenced", "20", "Death", "85", "20", "30",
"35", "Death", "Life", "25", "Probation", "Life without parole",
"35", "Life without parole", "Death", "Death", "Death"), Death_Penalty = c(0,
0, 0, 0, 0, 1, 0, 0, 0, 0, 1, 0, 0, 0, 0, 0, 0, 1, 1, 1), DNA_Only = c(0,
0, 0, 0, 0, 0, 0, 0, 0, 0, 0, 1, 0, 0, 0, 1, 1, 0, 0, 0), FC = c(1,
0, 0, 0, 0, 0, 0, 0, 0, 0, 0, 0, 0, 0, 0, 0, 0, 0, 0, 0), MWID = c(0,
0, 0, 0, 0, 0, 0, 1, 1, 1, 1, 1, 0, 0, 0, 1, 1, 0, 0, 0), F_MFE = c(0,
0, 1, 0, 0, 0, 0, 0, 0, 0, 1, 0, 0, 0, 0, 0, 0, 0, 0, 1), P_FA = c(1,
1, 1, 1, 1, 0, 1, 0, 0, 0, 0, 0, 1, 0, 0, 0, 0, 1, 0, 0), OM = c(1,
1, 1, 0, 1, 0, 1, 0, 0, 0, 0, 0, 0, 0, 0, 0, 0, 1, 1, 1), ILD = c(0,
0, 1, 0, 0, 1, 0, 0, 0, 0, 1, 0, 1, 0, 1, 0, 0, 0, 0, 0), State_Statute = c("Y",
"Y", "Y", "Y", "Y", "Y", "Y", "Y", "Y", "Y", "Y", "Y", "Y", "Y",
"Y", "Y", "Y", "Y", "Y", "Y"), State_Claim_Made = c(0, 0, 1,
0, 1, 0, 1, 0, 1, 0, 1, 0, 0, 0, 1, 0, 1, 0, 1 0), Zero_time = c(0,
0, 0, 1, 0, 0, 0, 0, 0, 0, 0, 0, 0, 1, 0, 0, 0, 0, 0, 0), Prem = c(0,
0, 0, 0, 0, 0, 0, 0, 0, 0, 0, 0, 0, 0, 0, 0, 0, 0, 0, 0), Pending = c(0,
0, 1, 0, 0, 0, 0, 0, 0, 0, 1, 0, 0, 0, 0, 0, 0, 0, 0, 0), Denied = c(0,
0, 0, 0, 0, 0, 1, 0, 0, 0, 0, 0, 0, 0, 0, 0, 0, 0, 0, 0), State_Award = c("0",
"0", "2", "0", "1", "0", "0", "0", "1", "0", "2", "0", "0", "0",
"0", "0", "0", "0", "0", "0"), Amount = c("0", "0", NA, "0",
"129041.88", "0", "0", "0", "1000000", "0", NA, "0", "0", "0",
"0", "0", "0", "0", "0", "0"), `Non-Statutory_Case_Filed` = c(0,
0, 0, 0, 0, 0, 0, 0, 0, 0, 0, 0, 0, 0, 0, 0, 0, 1, 1, 0), No_Time = c(0,
0, 0, 1, 0, 0, 0, 0, 0, 0, 0, 0, 0, 1, 0, 0, 0, 0, 0, 0), Unfiled = c(1,
1, 1, 1, 1, 1, 1, 1, 1, 1, 1, 1, 1, 1, 1, 1, 1, 0, 0, 1), Dismissed = c(0,
0, 0, 0, 0, 0, 0, 0, 0, 0, 0, 0, 0, 0, 0, 0, 0, 0, 1, 0), Pending__1 = c(0,
0, 0, 0, 0, 0, 0, 0, 0, 0, 0, 0, 0, 0, 0, 0, 0, 0, 0, 0), Award = c(0,
0, 0, 0, 0, 0, 0, 0, 0, 0, 0, 0, 0, 0, 0, 0, 0, 1, 0, 0), Premature = c(0,
0, 0, 0, 0, 0, 0, 0, 0, 0, 0, 0, 0, 0, 0, 0, 0, 0, 0, 0), Amount__1 = c("0",
"0", "0", "0", "0", "0", "0", "0", "0", "0", "0", "0", "0", "0",
"0", "0", "0", "$ undisclosed", "0", "0"), Years_Lost = c(1.7,
0.1, 19.5, 0, 2.6, 5.7, 1.8, 4, 10.7, 1.5, 28.5, 10.6, 10.1,
0, 5.8, 11.4, 11.4, 4.5, 5.4, 5.5), State_Award2 = c("0", "0",
"0", "0", "1", "0", "0", "0", "1", "0", "0", "0", "0", "0", "0",
"0", "0", "0", "0", "0")), row.names = c(NA, -20L), class = c("tbl_df",
"tbl", "data.frame"))
r ggplot2 categorical-data
r ggplot2 categorical-data
asked Nov 23 '18 at 22:47


Juanito TomasJuanito Tomas
111
111
migrated from stackoverflow.com Nov 24 '18 at 10:20
This question came from our site for professional and enthusiast programmers.
migrated from stackoverflow.com Nov 24 '18 at 10:20
This question came from our site for professional and enthusiast programmers.
add a comment |
add a comment |
1 Answer
1
active
oldest
votes
You can still use a logistic model with a categorical predictor:
g1 <- glm(State_Claim_Made ~ Race, family=binomial, data=jail)
summary(g1)
## Coefficients:
## Estimate Std. Error z value Pr(>|z|)
## (Intercept) -2.056e-16 7.071e-01 0.000 1.000
## RaceCaucasian -6.931e-01 9.354e-01 -0.741 0.459
In this case the log-odds difference between baseline (Black) and
Caucasian is -0.693 (log-odds of State_Claim_Made is lower
for Caucasian), but not significantly <0. For a sample size this small, the results of a GLM need to be interpreted very cautiously ...
Or you can use a contingency table approach:
(tt <- with(jail, table(Race,State_Claim_Made))
## State_Claim_Made
## Race 0 1
## Black 4 4
## Caucasian 8 4
chisq.test(tt, simulate.p.value=TRUE)
## data: tt
## X-squared = 0.55556, df = NA, p-value = 0.6432
I prefer the GLM as it's more flexible and gives you an effect size, but the contingency table analysis might be preferred for simplicity (and accuracy for small sample sizes).
Hmmmm, I see what you did here but how exactly would the coefficients explain the relationship between a race and their individual liklihood to see state claims? @Ben_Bolker
– Juanito Tomas
Nov 23 '18 at 23:45
Also, how do you justify doing a contingency table over an ANOVA test?
– Juanito Tomas
Nov 26 '18 at 23:35
add a comment |
Your Answer
StackExchange.ifUsing("editor", function () {
return StackExchange.using("mathjaxEditing", function () {
StackExchange.MarkdownEditor.creationCallbacks.add(function (editor, postfix) {
StackExchange.mathjaxEditing.prepareWmdForMathJax(editor, postfix, [["$", "$"], ["\\(","\\)"]]);
});
});
}, "mathjax-editing");
StackExchange.ready(function() {
var channelOptions = {
tags: "".split(" "),
id: "65"
};
initTagRenderer("".split(" "), "".split(" "), channelOptions);
StackExchange.using("externalEditor", function() {
// Have to fire editor after snippets, if snippets enabled
if (StackExchange.settings.snippets.snippetsEnabled) {
StackExchange.using("snippets", function() {
createEditor();
});
}
else {
createEditor();
}
});
function createEditor() {
StackExchange.prepareEditor({
heartbeatType: 'answer',
autoActivateHeartbeat: false,
convertImagesToLinks: false,
noModals: true,
showLowRepImageUploadWarning: true,
reputationToPostImages: null,
bindNavPrevention: true,
postfix: "",
imageUploader: {
brandingHtml: "Powered by u003ca class="icon-imgur-white" href="https://imgur.com/"u003eu003c/au003e",
contentPolicyHtml: "User contributions licensed under u003ca href="https://creativecommons.org/licenses/by-sa/3.0/"u003ecc by-sa 3.0 with attribution requiredu003c/au003e u003ca href="https://stackoverflow.com/legal/content-policy"u003e(content policy)u003c/au003e",
allowUrls: true
},
onDemand: true,
discardSelector: ".discard-answer"
,immediatelyShowMarkdownHelp:true
});
}
});
Sign up or log in
StackExchange.ready(function () {
StackExchange.helpers.onClickDraftSave('#login-link');
});
Sign up using Google
Sign up using Facebook
Sign up using Email and Password
Post as a guest
Required, but never shown
StackExchange.ready(
function () {
StackExchange.openid.initPostLogin('.new-post-login', 'https%3a%2f%2fstats.stackexchange.com%2fquestions%2f378529%2frelationship-between-a-categorical-variable-and-a-bernoulli-variable-in-r%23new-answer', 'question_page');
}
);
Post as a guest
Required, but never shown
1 Answer
1
active
oldest
votes
1 Answer
1
active
oldest
votes
active
oldest
votes
active
oldest
votes
You can still use a logistic model with a categorical predictor:
g1 <- glm(State_Claim_Made ~ Race, family=binomial, data=jail)
summary(g1)
## Coefficients:
## Estimate Std. Error z value Pr(>|z|)
## (Intercept) -2.056e-16 7.071e-01 0.000 1.000
## RaceCaucasian -6.931e-01 9.354e-01 -0.741 0.459
In this case the log-odds difference between baseline (Black) and
Caucasian is -0.693 (log-odds of State_Claim_Made is lower
for Caucasian), but not significantly <0. For a sample size this small, the results of a GLM need to be interpreted very cautiously ...
Or you can use a contingency table approach:
(tt <- with(jail, table(Race,State_Claim_Made))
## State_Claim_Made
## Race 0 1
## Black 4 4
## Caucasian 8 4
chisq.test(tt, simulate.p.value=TRUE)
## data: tt
## X-squared = 0.55556, df = NA, p-value = 0.6432
I prefer the GLM as it's more flexible and gives you an effect size, but the contingency table analysis might be preferred for simplicity (and accuracy for small sample sizes).
Hmmmm, I see what you did here but how exactly would the coefficients explain the relationship between a race and their individual liklihood to see state claims? @Ben_Bolker
– Juanito Tomas
Nov 23 '18 at 23:45
Also, how do you justify doing a contingency table over an ANOVA test?
– Juanito Tomas
Nov 26 '18 at 23:35
add a comment |
You can still use a logistic model with a categorical predictor:
g1 <- glm(State_Claim_Made ~ Race, family=binomial, data=jail)
summary(g1)
## Coefficients:
## Estimate Std. Error z value Pr(>|z|)
## (Intercept) -2.056e-16 7.071e-01 0.000 1.000
## RaceCaucasian -6.931e-01 9.354e-01 -0.741 0.459
In this case the log-odds difference between baseline (Black) and
Caucasian is -0.693 (log-odds of State_Claim_Made is lower
for Caucasian), but not significantly <0. For a sample size this small, the results of a GLM need to be interpreted very cautiously ...
Or you can use a contingency table approach:
(tt <- with(jail, table(Race,State_Claim_Made))
## State_Claim_Made
## Race 0 1
## Black 4 4
## Caucasian 8 4
chisq.test(tt, simulate.p.value=TRUE)
## data: tt
## X-squared = 0.55556, df = NA, p-value = 0.6432
I prefer the GLM as it's more flexible and gives you an effect size, but the contingency table analysis might be preferred for simplicity (and accuracy for small sample sizes).
Hmmmm, I see what you did here but how exactly would the coefficients explain the relationship between a race and their individual liklihood to see state claims? @Ben_Bolker
– Juanito Tomas
Nov 23 '18 at 23:45
Also, how do you justify doing a contingency table over an ANOVA test?
– Juanito Tomas
Nov 26 '18 at 23:35
add a comment |
You can still use a logistic model with a categorical predictor:
g1 <- glm(State_Claim_Made ~ Race, family=binomial, data=jail)
summary(g1)
## Coefficients:
## Estimate Std. Error z value Pr(>|z|)
## (Intercept) -2.056e-16 7.071e-01 0.000 1.000
## RaceCaucasian -6.931e-01 9.354e-01 -0.741 0.459
In this case the log-odds difference between baseline (Black) and
Caucasian is -0.693 (log-odds of State_Claim_Made is lower
for Caucasian), but not significantly <0. For a sample size this small, the results of a GLM need to be interpreted very cautiously ...
Or you can use a contingency table approach:
(tt <- with(jail, table(Race,State_Claim_Made))
## State_Claim_Made
## Race 0 1
## Black 4 4
## Caucasian 8 4
chisq.test(tt, simulate.p.value=TRUE)
## data: tt
## X-squared = 0.55556, df = NA, p-value = 0.6432
I prefer the GLM as it's more flexible and gives you an effect size, but the contingency table analysis might be preferred for simplicity (and accuracy for small sample sizes).
You can still use a logistic model with a categorical predictor:
g1 <- glm(State_Claim_Made ~ Race, family=binomial, data=jail)
summary(g1)
## Coefficients:
## Estimate Std. Error z value Pr(>|z|)
## (Intercept) -2.056e-16 7.071e-01 0.000 1.000
## RaceCaucasian -6.931e-01 9.354e-01 -0.741 0.459
In this case the log-odds difference between baseline (Black) and
Caucasian is -0.693 (log-odds of State_Claim_Made is lower
for Caucasian), but not significantly <0. For a sample size this small, the results of a GLM need to be interpreted very cautiously ...
Or you can use a contingency table approach:
(tt <- with(jail, table(Race,State_Claim_Made))
## State_Claim_Made
## Race 0 1
## Black 4 4
## Caucasian 8 4
chisq.test(tt, simulate.p.value=TRUE)
## data: tt
## X-squared = 0.55556, df = NA, p-value = 0.6432
I prefer the GLM as it's more flexible and gives you an effect size, but the contingency table analysis might be preferred for simplicity (and accuracy for small sample sizes).
edited Nov 27 '18 at 15:17
answered Nov 23 '18 at 23:02
Ben BolkerBen Bolker
22.8k16191
22.8k16191
Hmmmm, I see what you did here but how exactly would the coefficients explain the relationship between a race and their individual liklihood to see state claims? @Ben_Bolker
– Juanito Tomas
Nov 23 '18 at 23:45
Also, how do you justify doing a contingency table over an ANOVA test?
– Juanito Tomas
Nov 26 '18 at 23:35
add a comment |
Hmmmm, I see what you did here but how exactly would the coefficients explain the relationship between a race and their individual liklihood to see state claims? @Ben_Bolker
– Juanito Tomas
Nov 23 '18 at 23:45
Also, how do you justify doing a contingency table over an ANOVA test?
– Juanito Tomas
Nov 26 '18 at 23:35
Hmmmm, I see what you did here but how exactly would the coefficients explain the relationship between a race and their individual liklihood to see state claims? @Ben_Bolker
– Juanito Tomas
Nov 23 '18 at 23:45
Hmmmm, I see what you did here but how exactly would the coefficients explain the relationship between a race and their individual liklihood to see state claims? @Ben_Bolker
– Juanito Tomas
Nov 23 '18 at 23:45
Also, how do you justify doing a contingency table over an ANOVA test?
– Juanito Tomas
Nov 26 '18 at 23:35
Also, how do you justify doing a contingency table over an ANOVA test?
– Juanito Tomas
Nov 26 '18 at 23:35
add a comment |
Thanks for contributing an answer to Cross Validated!
- Please be sure to answer the question. Provide details and share your research!
But avoid …
- Asking for help, clarification, or responding to other answers.
- Making statements based on opinion; back them up with references or personal experience.
Use MathJax to format equations. MathJax reference.
To learn more, see our tips on writing great answers.
Sign up or log in
StackExchange.ready(function () {
StackExchange.helpers.onClickDraftSave('#login-link');
});
Sign up using Google
Sign up using Facebook
Sign up using Email and Password
Post as a guest
Required, but never shown
StackExchange.ready(
function () {
StackExchange.openid.initPostLogin('.new-post-login', 'https%3a%2f%2fstats.stackexchange.com%2fquestions%2f378529%2frelationship-between-a-categorical-variable-and-a-bernoulli-variable-in-r%23new-answer', 'question_page');
}
);
Post as a guest
Required, but never shown
Sign up or log in
StackExchange.ready(function () {
StackExchange.helpers.onClickDraftSave('#login-link');
});
Sign up using Google
Sign up using Facebook
Sign up using Email and Password
Post as a guest
Required, but never shown
Sign up or log in
StackExchange.ready(function () {
StackExchange.helpers.onClickDraftSave('#login-link');
});
Sign up using Google
Sign up using Facebook
Sign up using Email and Password
Post as a guest
Required, but never shown
Sign up or log in
StackExchange.ready(function () {
StackExchange.helpers.onClickDraftSave('#login-link');
});
Sign up using Google
Sign up using Facebook
Sign up using Email and Password
Sign up using Google
Sign up using Facebook
Sign up using Email and Password
Post as a guest
Required, but never shown
Required, but never shown
Required, but never shown
Required, but never shown
Required, but never shown
Required, but never shown
Required, but never shown
Required, but never shown
Required, but never shown
zPbSp8B6pwfma,rpUt4yzR YLFO3KjXJTtHxoTjPcx7JdZLmMg7I8Ls7ccGqh2Hk,FJ5GJ62 vW8CXYIX