Load huge image dataset and some data augmentation using Pytorch
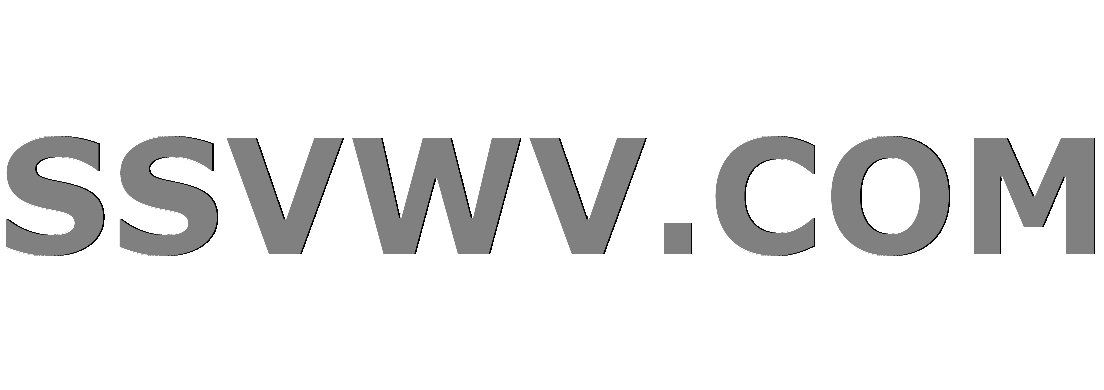
Multi tool use
I have a question. I have about 2 million images (place365-standard dataset) and I want to do some data augmentation like transforming, cropping etc. Also, I have to make my own target image (y) based on some color model algorithms (CMYK) for example.
So Actually, my preprocessing step includes augmentation and making terget image (y). And then I should feed these images to a deep network. When should I do this based on Dataset
class? Should I do my processing step in __getitem__()
? If yes, would it be parallel and fast?
Here is my template of Dataset(data.Dataset)
class:
import torch
from torch.utils import data
class Dataset(data.Dataset):
"""
Return Dataset class representing our data set
"""
def __int__(self, list_IDs, labels):
"""
Initialize data set as a list of IDs corresponding to each item of data set and labels of each data
Args:
list_IDs: a list of IDs for each data point in data set
labels: label of an item in data set with respect to the ID
"""
self.labels = labels
self.list_IDs = list_IDs
def __len__(self):
"""
Return the length of data set using list of IDs
:return: number of samples in data set
"""
return len(self.list_IDs)
def __getitem__(self, item):
"""
Generate one item of data set. Here we apply our preprocessing things like halftone styles and subtractive color process using CMYK color model etc. (See the paper for operations)
:param item: index of item in IDs list
:return: a sample of data
"""
ID = self.list_IDs[item]
# Code to load data
X = None #
# code to apply your custom function to make y image (time consuming task - some algorithms)
y = None #
return X, y
Thanks for any advice
Best regards
python image-processing deep-learning bigdata pytorch
add a comment |
I have a question. I have about 2 million images (place365-standard dataset) and I want to do some data augmentation like transforming, cropping etc. Also, I have to make my own target image (y) based on some color model algorithms (CMYK) for example.
So Actually, my preprocessing step includes augmentation and making terget image (y). And then I should feed these images to a deep network. When should I do this based on Dataset
class? Should I do my processing step in __getitem__()
? If yes, would it be parallel and fast?
Here is my template of Dataset(data.Dataset)
class:
import torch
from torch.utils import data
class Dataset(data.Dataset):
"""
Return Dataset class representing our data set
"""
def __int__(self, list_IDs, labels):
"""
Initialize data set as a list of IDs corresponding to each item of data set and labels of each data
Args:
list_IDs: a list of IDs for each data point in data set
labels: label of an item in data set with respect to the ID
"""
self.labels = labels
self.list_IDs = list_IDs
def __len__(self):
"""
Return the length of data set using list of IDs
:return: number of samples in data set
"""
return len(self.list_IDs)
def __getitem__(self, item):
"""
Generate one item of data set. Here we apply our preprocessing things like halftone styles and subtractive color process using CMYK color model etc. (See the paper for operations)
:param item: index of item in IDs list
:return: a sample of data
"""
ID = self.list_IDs[item]
# Code to load data
X = None #
# code to apply your custom function to make y image (time consuming task - some algorithms)
y = None #
return X, y
Thanks for any advice
Best regards
python image-processing deep-learning bigdata pytorch
add a comment |
I have a question. I have about 2 million images (place365-standard dataset) and I want to do some data augmentation like transforming, cropping etc. Also, I have to make my own target image (y) based on some color model algorithms (CMYK) for example.
So Actually, my preprocessing step includes augmentation and making terget image (y). And then I should feed these images to a deep network. When should I do this based on Dataset
class? Should I do my processing step in __getitem__()
? If yes, would it be parallel and fast?
Here is my template of Dataset(data.Dataset)
class:
import torch
from torch.utils import data
class Dataset(data.Dataset):
"""
Return Dataset class representing our data set
"""
def __int__(self, list_IDs, labels):
"""
Initialize data set as a list of IDs corresponding to each item of data set and labels of each data
Args:
list_IDs: a list of IDs for each data point in data set
labels: label of an item in data set with respect to the ID
"""
self.labels = labels
self.list_IDs = list_IDs
def __len__(self):
"""
Return the length of data set using list of IDs
:return: number of samples in data set
"""
return len(self.list_IDs)
def __getitem__(self, item):
"""
Generate one item of data set. Here we apply our preprocessing things like halftone styles and subtractive color process using CMYK color model etc. (See the paper for operations)
:param item: index of item in IDs list
:return: a sample of data
"""
ID = self.list_IDs[item]
# Code to load data
X = None #
# code to apply your custom function to make y image (time consuming task - some algorithms)
y = None #
return X, y
Thanks for any advice
Best regards
python image-processing deep-learning bigdata pytorch
I have a question. I have about 2 million images (place365-standard dataset) and I want to do some data augmentation like transforming, cropping etc. Also, I have to make my own target image (y) based on some color model algorithms (CMYK) for example.
So Actually, my preprocessing step includes augmentation and making terget image (y). And then I should feed these images to a deep network. When should I do this based on Dataset
class? Should I do my processing step in __getitem__()
? If yes, would it be parallel and fast?
Here is my template of Dataset(data.Dataset)
class:
import torch
from torch.utils import data
class Dataset(data.Dataset):
"""
Return Dataset class representing our data set
"""
def __int__(self, list_IDs, labels):
"""
Initialize data set as a list of IDs corresponding to each item of data set and labels of each data
Args:
list_IDs: a list of IDs for each data point in data set
labels: label of an item in data set with respect to the ID
"""
self.labels = labels
self.list_IDs = list_IDs
def __len__(self):
"""
Return the length of data set using list of IDs
:return: number of samples in data set
"""
return len(self.list_IDs)
def __getitem__(self, item):
"""
Generate one item of data set. Here we apply our preprocessing things like halftone styles and subtractive color process using CMYK color model etc. (See the paper for operations)
:param item: index of item in IDs list
:return: a sample of data
"""
ID = self.list_IDs[item]
# Code to load data
X = None #
# code to apply your custom function to make y image (time consuming task - some algorithms)
y = None #
return X, y
Thanks for any advice
Best regards
python image-processing deep-learning bigdata pytorch
python image-processing deep-learning bigdata pytorch
edited Nov 24 '18 at 6:40
M. Doosti Lakhani
asked Nov 23 '18 at 22:52


M. Doosti LakhaniM. Doosti Lakhani
324317
324317
add a comment |
add a comment |
1 Answer
1
active
oldest
votes
If you look at e.g., torchvision.dataset.ImageFolder
you'll see that it works quite similar to your design: the class has transform
member that lists all sorts of augmentations (resizing, cropping, flipping etc.) and these are carried out on the images in the __getitem__
method.
Regarding parallelism, the Dataset
itself is not parallel, but the DataLoader
can be (see num_workers
argument), so if you use your dataset inside a parallel dataloader you get the parallelism for free, Cool!
Thank you. But i have a problem. I have a 101GB.tar
file contains about 1.8 million images(place365-standard), now I have to do some preprocessing like you mentioned (transforms). But I have to do something else here. I should apply some algorithms to generate new images as ground-truth (y). But I do not know where should I put this codes. Should I put my codes in__getitem__()
function? Actually, I should consider RAM size, and I should do everything parallel (on GPU and CPU). I do not anything and I am new to this type of implementation.
– M. Doosti Lakhani
Nov 24 '18 at 18:32
@M.DoostiLakhani how does y relates to x?
– Shai
Nov 24 '18 at 18:33
I have to do some color model algorithms like CMYK to generate halftone image for printing or scanning applications.
– M. Doosti Lakhani
Nov 24 '18 at 19:14
add a comment |
Your Answer
StackExchange.ifUsing("editor", function () {
StackExchange.using("externalEditor", function () {
StackExchange.using("snippets", function () {
StackExchange.snippets.init();
});
});
}, "code-snippets");
StackExchange.ready(function() {
var channelOptions = {
tags: "".split(" "),
id: "1"
};
initTagRenderer("".split(" "), "".split(" "), channelOptions);
StackExchange.using("externalEditor", function() {
// Have to fire editor after snippets, if snippets enabled
if (StackExchange.settings.snippets.snippetsEnabled) {
StackExchange.using("snippets", function() {
createEditor();
});
}
else {
createEditor();
}
});
function createEditor() {
StackExchange.prepareEditor({
heartbeatType: 'answer',
autoActivateHeartbeat: false,
convertImagesToLinks: true,
noModals: true,
showLowRepImageUploadWarning: true,
reputationToPostImages: 10,
bindNavPrevention: true,
postfix: "",
imageUploader: {
brandingHtml: "Powered by u003ca class="icon-imgur-white" href="https://imgur.com/"u003eu003c/au003e",
contentPolicyHtml: "User contributions licensed under u003ca href="https://creativecommons.org/licenses/by-sa/3.0/"u003ecc by-sa 3.0 with attribution requiredu003c/au003e u003ca href="https://stackoverflow.com/legal/content-policy"u003e(content policy)u003c/au003e",
allowUrls: true
},
onDemand: true,
discardSelector: ".discard-answer"
,immediatelyShowMarkdownHelp:true
});
}
});
Sign up or log in
StackExchange.ready(function () {
StackExchange.helpers.onClickDraftSave('#login-link');
});
Sign up using Google
Sign up using Facebook
Sign up using Email and Password
Post as a guest
Required, but never shown
StackExchange.ready(
function () {
StackExchange.openid.initPostLogin('.new-post-login', 'https%3a%2f%2fstackoverflow.com%2fquestions%2f53453663%2fload-huge-image-dataset-and-some-data-augmentation-using-pytorch%23new-answer', 'question_page');
}
);
Post as a guest
Required, but never shown
1 Answer
1
active
oldest
votes
1 Answer
1
active
oldest
votes
active
oldest
votes
active
oldest
votes
If you look at e.g., torchvision.dataset.ImageFolder
you'll see that it works quite similar to your design: the class has transform
member that lists all sorts of augmentations (resizing, cropping, flipping etc.) and these are carried out on the images in the __getitem__
method.
Regarding parallelism, the Dataset
itself is not parallel, but the DataLoader
can be (see num_workers
argument), so if you use your dataset inside a parallel dataloader you get the parallelism for free, Cool!
Thank you. But i have a problem. I have a 101GB.tar
file contains about 1.8 million images(place365-standard), now I have to do some preprocessing like you mentioned (transforms). But I have to do something else here. I should apply some algorithms to generate new images as ground-truth (y). But I do not know where should I put this codes. Should I put my codes in__getitem__()
function? Actually, I should consider RAM size, and I should do everything parallel (on GPU and CPU). I do not anything and I am new to this type of implementation.
– M. Doosti Lakhani
Nov 24 '18 at 18:32
@M.DoostiLakhani how does y relates to x?
– Shai
Nov 24 '18 at 18:33
I have to do some color model algorithms like CMYK to generate halftone image for printing or scanning applications.
– M. Doosti Lakhani
Nov 24 '18 at 19:14
add a comment |
If you look at e.g., torchvision.dataset.ImageFolder
you'll see that it works quite similar to your design: the class has transform
member that lists all sorts of augmentations (resizing, cropping, flipping etc.) and these are carried out on the images in the __getitem__
method.
Regarding parallelism, the Dataset
itself is not parallel, but the DataLoader
can be (see num_workers
argument), so if you use your dataset inside a parallel dataloader you get the parallelism for free, Cool!
Thank you. But i have a problem. I have a 101GB.tar
file contains about 1.8 million images(place365-standard), now I have to do some preprocessing like you mentioned (transforms). But I have to do something else here. I should apply some algorithms to generate new images as ground-truth (y). But I do not know where should I put this codes. Should I put my codes in__getitem__()
function? Actually, I should consider RAM size, and I should do everything parallel (on GPU and CPU). I do not anything and I am new to this type of implementation.
– M. Doosti Lakhani
Nov 24 '18 at 18:32
@M.DoostiLakhani how does y relates to x?
– Shai
Nov 24 '18 at 18:33
I have to do some color model algorithms like CMYK to generate halftone image for printing or scanning applications.
– M. Doosti Lakhani
Nov 24 '18 at 19:14
add a comment |
If you look at e.g., torchvision.dataset.ImageFolder
you'll see that it works quite similar to your design: the class has transform
member that lists all sorts of augmentations (resizing, cropping, flipping etc.) and these are carried out on the images in the __getitem__
method.
Regarding parallelism, the Dataset
itself is not parallel, but the DataLoader
can be (see num_workers
argument), so if you use your dataset inside a parallel dataloader you get the parallelism for free, Cool!
If you look at e.g., torchvision.dataset.ImageFolder
you'll see that it works quite similar to your design: the class has transform
member that lists all sorts of augmentations (resizing, cropping, flipping etc.) and these are carried out on the images in the __getitem__
method.
Regarding parallelism, the Dataset
itself is not parallel, but the DataLoader
can be (see num_workers
argument), so if you use your dataset inside a parallel dataloader you get the parallelism for free, Cool!
answered Nov 24 '18 at 18:03
ShaiShai
69.3k22135242
69.3k22135242
Thank you. But i have a problem. I have a 101GB.tar
file contains about 1.8 million images(place365-standard), now I have to do some preprocessing like you mentioned (transforms). But I have to do something else here. I should apply some algorithms to generate new images as ground-truth (y). But I do not know where should I put this codes. Should I put my codes in__getitem__()
function? Actually, I should consider RAM size, and I should do everything parallel (on GPU and CPU). I do not anything and I am new to this type of implementation.
– M. Doosti Lakhani
Nov 24 '18 at 18:32
@M.DoostiLakhani how does y relates to x?
– Shai
Nov 24 '18 at 18:33
I have to do some color model algorithms like CMYK to generate halftone image for printing or scanning applications.
– M. Doosti Lakhani
Nov 24 '18 at 19:14
add a comment |
Thank you. But i have a problem. I have a 101GB.tar
file contains about 1.8 million images(place365-standard), now I have to do some preprocessing like you mentioned (transforms). But I have to do something else here. I should apply some algorithms to generate new images as ground-truth (y). But I do not know where should I put this codes. Should I put my codes in__getitem__()
function? Actually, I should consider RAM size, and I should do everything parallel (on GPU and CPU). I do not anything and I am new to this type of implementation.
– M. Doosti Lakhani
Nov 24 '18 at 18:32
@M.DoostiLakhani how does y relates to x?
– Shai
Nov 24 '18 at 18:33
I have to do some color model algorithms like CMYK to generate halftone image for printing or scanning applications.
– M. Doosti Lakhani
Nov 24 '18 at 19:14
Thank you. But i have a problem. I have a 101GB
.tar
file contains about 1.8 million images(place365-standard), now I have to do some preprocessing like you mentioned (transforms). But I have to do something else here. I should apply some algorithms to generate new images as ground-truth (y). But I do not know where should I put this codes. Should I put my codes in __getitem__()
function? Actually, I should consider RAM size, and I should do everything parallel (on GPU and CPU). I do not anything and I am new to this type of implementation.– M. Doosti Lakhani
Nov 24 '18 at 18:32
Thank you. But i have a problem. I have a 101GB
.tar
file contains about 1.8 million images(place365-standard), now I have to do some preprocessing like you mentioned (transforms). But I have to do something else here. I should apply some algorithms to generate new images as ground-truth (y). But I do not know where should I put this codes. Should I put my codes in __getitem__()
function? Actually, I should consider RAM size, and I should do everything parallel (on GPU and CPU). I do not anything and I am new to this type of implementation.– M. Doosti Lakhani
Nov 24 '18 at 18:32
@M.DoostiLakhani how does y relates to x?
– Shai
Nov 24 '18 at 18:33
@M.DoostiLakhani how does y relates to x?
– Shai
Nov 24 '18 at 18:33
I have to do some color model algorithms like CMYK to generate halftone image for printing or scanning applications.
– M. Doosti Lakhani
Nov 24 '18 at 19:14
I have to do some color model algorithms like CMYK to generate halftone image for printing or scanning applications.
– M. Doosti Lakhani
Nov 24 '18 at 19:14
add a comment |
Thanks for contributing an answer to Stack Overflow!
- Please be sure to answer the question. Provide details and share your research!
But avoid …
- Asking for help, clarification, or responding to other answers.
- Making statements based on opinion; back them up with references or personal experience.
To learn more, see our tips on writing great answers.
Sign up or log in
StackExchange.ready(function () {
StackExchange.helpers.onClickDraftSave('#login-link');
});
Sign up using Google
Sign up using Facebook
Sign up using Email and Password
Post as a guest
Required, but never shown
StackExchange.ready(
function () {
StackExchange.openid.initPostLogin('.new-post-login', 'https%3a%2f%2fstackoverflow.com%2fquestions%2f53453663%2fload-huge-image-dataset-and-some-data-augmentation-using-pytorch%23new-answer', 'question_page');
}
);
Post as a guest
Required, but never shown
Sign up or log in
StackExchange.ready(function () {
StackExchange.helpers.onClickDraftSave('#login-link');
});
Sign up using Google
Sign up using Facebook
Sign up using Email and Password
Post as a guest
Required, but never shown
Sign up or log in
StackExchange.ready(function () {
StackExchange.helpers.onClickDraftSave('#login-link');
});
Sign up using Google
Sign up using Facebook
Sign up using Email and Password
Post as a guest
Required, but never shown
Sign up or log in
StackExchange.ready(function () {
StackExchange.helpers.onClickDraftSave('#login-link');
});
Sign up using Google
Sign up using Facebook
Sign up using Email and Password
Sign up using Google
Sign up using Facebook
Sign up using Email and Password
Post as a guest
Required, but never shown
Required, but never shown
Required, but never shown
Required, but never shown
Required, but never shown
Required, but never shown
Required, but never shown
Required, but never shown
Required, but never shown
P6HuUXWCeWrOnY y9cC0 0E cZwY,1EGUIN8K R